Regression models for the value of rice production in Rio Grande do Sul
Keywords:
Gamma distribution, generalized linear model, linear model, riceAbstract
Rice is the second most consumed grain in the world. In Brazil, Rio Grande do Sul (RS) is the largest producer of paddy rice. Therefore, the objective of this study is to evaluate the factors that influence the production value of this cereal. To explain the value of rice production, production variables obtained from the Department of Economics and Statistics website and derived variables taken from the Brazilian Daily Weather Gridded Data website were used, referring to the year 2019. The variables found present a non-normal and heteroscedastic distribution, justifying the use of the generalized linear regression technique. The selection of variables for the models was done using the stepwise method. The models found demonstrated that the annual average of solar radiation and the annual average of rainfall transfer influence the value of rice production in RS, as well as in other studies. However, these models may not be suitable to capture the non-linear relationship between grain production value and variables such as climate and production.
References
ATLAS. Arroz: O Rio Grande do Sul é o maior produtor de arroz em casca do Brasil. 7ª ed. 2022. Acesso em: 04 de out. 2022. Disponível em: https://atlassocioeconomico.rs.gov.br/arroz
CORDEIRO, G. M.; DEMÉTRIO, C. G. Modelos Lineares Generalizados. Minicurso para o 12º SEAGRO e a 52ª Reunião Anual da RBRAS UFSM, Santa Maria, RS,2012. Disponível em: https://docs.ufpr.br/~lucambio/%20CE225/2S2009/livroSeagro.pdf
DA SILVA, E. V.; JACOBI, L. F. Regressão linear e não linear aplicado ao estudo de casos de AIDS na Região Norte do Brasil. Ciência E Natura, v. 42, e27, 2020. https://doi.org/10.5902/2179460X40535
DEEDADOS (2023). Departamento de economia e estatística dados abertos. Disponível em: https://dados.fee.tche.br/
EKANAYAKE, E., WICKRAMASINGHE, L., WELIWATTA, R. Use of regression techniques for rice yield estimation in the north-western province of sri lanka. Ceylon journal of science, v. 50, n. 4, p. 439–447, 2021. https://doi.org/10.4038/cjs.v50i4.7942
MATEUS, W. S.; MATEUS, A. L. S. S.; BUENO FILHO, J. S. DE S. Avaliação por regressão linear múltipla para valor de imóveis residenciais. Sigmae, v. 8, n. 2, p. 784–787, 2019. https://publicacoes.unifal-mg.edu.br/revistas/index.php/sigmae/article/view/987
MCCULLAGH, P.; NELDER, J. A. Generalized linear models, 2nd edidion. Chapman and Hall, London, 1989, 526 p.
MONTGOMERY, D. C; RUNGER, G. C. Estatística aplicada e probabilidade para engenheiros. 4a edição. Rio de Janeiro: LTC Editora, 2009, 514 p.
NUGRAHANI, I. D.; SUSANTI, Y.; QONA’AH, N. Modeling of rice production in indonesia using robust regression with the method of moments (mm) estimation. Nusantara science and technology proceedings, v. 21, p. 79–87, 2021. http://dx.doi.org/10.11594/nstp.2021.1111
R CORE TEAM.R: A language and environment for statistical computing. R Foundation forStatistical Computing, Vienna, Austria. 2022. Disponível em: https://www.r-project.org/
RIBEIRO et al. Soybean production value in the Rio Grande do Sul under the GAMLSS framework. Communications in statistics: case studies, data analysis and applications. v. 7, n. 2, p. 146-165, 2021. http://dx.doi.org /10.1080/23737484.2020.1852131
SILVA, L. E. M. DA; JACOBI, L. F.; LÚCIO, A. D. Analysis of cases of death due to stroke in the state of Rio Grande do Sul from 1979 to 2014. Sigmae, v. 8, n. 2, p. 274–281, 2019. https://publicacoes.unifal-mg.edu.br/revistas/index.php/sigmae/article/view/954
SILVIO STEINMETZ, J. B. D. S.; DEIBLER, N. A. Estimativa da produtividade de arroz irrigado em função da radiação solar global e da temperatura mínima do ar. Ciência Rural, Santa Maria, v. 43, n. 2, p. 206-211, 2013. https://doi.org/10.1590/S0103-84782013000200003
URRUTIA, J. D. et al. Forecasting rice production in luzon using integrated spatio-temporal forecasting framework. Em: AIP Conference Proceedings, AIP Publishing LLC, v. 2192, p. 09004, 2019. http://dx.doi.org /10.1063/1.5139184
WICKRAMASINGHE, L.; JAYASINGHE, J.; RATHNAYAKE, U. Relationships between climatic factors to the paddy yield in the north-western province of sri lanka. Em: International Research Conference on Smart Computing and Systems Engineering (SCSE), IEEE, p. 223–227, 2020. http://dx.doi.org /10.1109/SCSE49731.2020.9313047
XAVIER, A. C. Brazilian daily weather gridded data (br-dwgd), 2023. Disponível em: https://sites.google.com/site/alexandrecandidoxavierufes/brazilian-daily-weather-gridded-data
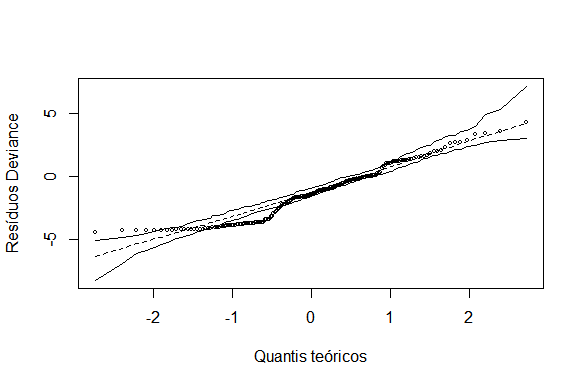
Downloads
Published
How to Cite
Issue
Section
License
Proposta de Política para Periódicos de Acesso Livre
Autores que publicam nesta revista concordam com os seguintes termos:
- Autores mantém os direitos autorais e concedem à revista o direito de primeira publicação, com o trabalho simultaneamente licenciado sob a Licença Creative Commons Attribution que permite o compartilhamento do trabalho com reconhecimento da autoria e publicação inicial nesta revista.
- Autores têm autorização para assumir contratos adicionais separadamente, para distribuição não-exclusiva da versão do trabalho publicada nesta revista (ex.: publicar em repositório institucional ou como capítulo de livro), com reconhecimento de autoria e publicação inicial nesta revista.
- Autores têm permissão e são estimulados a publicar e distribuir seu trabalho online (ex.: em repositórios institucionais ou na sua página pessoal) a qualquer ponto antes ou durante o processo editorial, já que isso pode gerar alterações produtivas, bem como aumentar o impacto e a citação do trabalho publicado (Veja O Efeito do Acesso Livre).