Transformação de dados sob heterocedasticidade e normalidade
um estudo de simulação
Palabras clave:
Poder do teste, nível de significância , pressuposiçõesResumen
A Análise de Variância (ANOVA) é uma das técnicas de inferência estatística mais utilizadas nas ciências biológicas, ecológicas e agrícolas e depende significativamente da pressuposição de variâncias homogêneas ao longo dos tratamentos. Pouco é conhecido sobre as propriedades do teste F com diferentes transformações sob normalidade e heterocedasticidade. Por isso, este estudo tem o objetivo de avaliar o impacto da transformação no poder e no nível de significância do teste F sob heterocedasticidade. Para isso, um estudo de simulação foi realizado utilizando 6 transformações, 3 níveis de desigualdade de variâncias, 7 repetições e 10.000 dados simulados. As proporções de médias 5-5-5 e 5-5-6 foram utilizadas para avaliar o nível de significância e o poder do teste, respectivamente. O teste de Bartlett foi realizado em cada dado simulado visando definir o Índice de Capacidade de Homogeneização (ICH) para cada transformação. Os resultados foram analisados usando o teste F da ANOVA ao nível de 5% de probabilidade e as médias foram comparadas pelo teste de Tukey, após a verificação das pressuposições. A transformação de dados não aumenta o poder do teste, não mantém o nível de significância e reduz o HCI para todos os níveis de heterocedasticidade. Uma vez que o ICH se mantém em torno de 40% após a aplicação das transformações, é possível obter resultados positivos em testes de pressuposições apesar da redução na qualidade do teste. O uso de transformações para lidar com a heterocedasticidade para dados normais não é uma estratégia efetiva e testes robustos devem ser utilizados.
Citas
ASLAM, Muhammad. Design of the Bartlett and Hartley tests for homogeneity of variances under
indeterminacy environment. Journal Of Taibah University For Science, [S.L.], v. 14, n. 1, p. 6-10, 10 dez.
Informa UK Limited. http://dx.doi.org/10.1080/16583655.2019.1700675.
BLANCA, María J.; ALARCÓN, Rafael; ARNAU, Jaume. Non-normal data: is anova still a valid option?.
Psicothema, [S.L.], n. 294, p. 552-557, nov. 2017. C.O.P. del Ppdo. de Asturias.
http://dx.doi.org/10.7334/psicothema2016.383.
BLANCA, María J.; ALARCÓN, Rafael; ARNAU, Jaume; BONO, Roser; BENDAYAN, Rebecca. Effect of
variance ratio on ANOVA robustness: might 1.5 be the limit?. Behavior Research Methods, [S.L.], v. 50, n.
, p. 937-962, 22 jun. 2017. Springer Science and Business Media LLC. http://dx.doi.org/10.3758/s13428-
-0918-2.
FERNANDEZ, George C.J.. Residual Analysis and Data Transformations: important tools in statistical
analysis. Hortscience, [S.L.], v. 27, n. 4, p. 297-300, abr. 1992. American Society for Horticultural Science.
http://dx.doi.org/10.21273/hortsci.27.4.297.
KARIYA, Takeaki; KURATA, Hiroshi. Generalized Least Squares. Wiley Series In Probability And
Statistics, [S.L.], p. 500-545, 25 jun. 2004. Wiley. http://dx.doi.org/10.1002/0470866993.
KNIEF, Ulrich; FORSTMEIER, Wolfgang. Violating the normality assumption may be the lesser of two evils.
Behavior Research Methods, [S.L.], v. 53, n. 6, p. 2576-2590, 7 maio 2021. Springer Science and Business
Media LLC. http://dx.doi.org/10.3758/s13428-021-01587-5.
Kozak, M. Analyzing one-way experiments: a piece of cake or a pain in the neck. Sci. Agric, 4, 556–562.
https://www.scielo.br/j/sa/a/6nsk8bJv9zsyWR7SbPfwmQG/?format=pdf.
MAHAPOONYANONT, Natcha; MAHAPOONYANONT, Tharadeth; PENGKAEW, Nussara;
KAMHANGKIT, Rojarek. Power of the test of One-Way Anova after transforming with large sample size
data. Procedia - Social And Behavioral Sciences, [S.L.], v. 9, p. 933-937, 2010. Elsevier BV.
http://dx.doi.org/10.1016/j.sbspro.2010.12.262.
MODER, K. Alternatives to F-test in one way ANOVA in case of heterogeneity of variances (a simulation
study). Psychological Test and Assessment Modeling, 52(4), 343–353, 2010.
MORRIS, Tim P.; WHITE, Ian R.; CROWTHER, Michael J.. Using simulation studies to evaluate statistical
methods. Statistics In Medicine, [S.L.], v. 38, n. 11, p. 2074-2102, 16 jan. 2019. Wiley.
http://dx.doi.org/10.1002/sim.8086.
OSBORNE, Jason. Notes on the use of data transformations. University Of Massachusetts Amherst, [S.L.],
p. 37-45, 2002. University of Massachusetts Amherst. http://dx.doi.org/10.7275/4VNG-5608.
PEK, J.; WONG, O.; WONG, A. C.. Data Transformations for Inference with Linear Regression: clarifications
and recommendations. University Of Massachusetts Amherst, [S.L.], p. 22-35, 2017. University of
Massachusetts Amherst. http://dx.doi.org/10.7275/2W3N-0F07.
SNEDECOR, G. W.. Statistical methods applied to experiments in agriculture and biology, 1st–5th editions.
Ames, IA: Collegiate Press, 1956.
STANTON, Maureen L.; THIEDE, Denise A.. Statistical convenience vs biological insight: consequences of
data transformation for the analysis of fitness variation in heterogeneous environments. New Phytologist,
[S.L.], v. 166, n. 1, p. 319-338, 12 jan. 2005. Wiley. http://dx.doi.org/10.1111/j.1469-8137.2004.01311.x.
ST‐PIERRE, Anne P.; SHIKON, Violaine; SCHNEIDER, David C.. Count data in biology—Data
transformation or model reformation? Ecology And Evolution, [S.L.], v. 8, n. 6, p. 3077-3085, 16 fev. 2018.
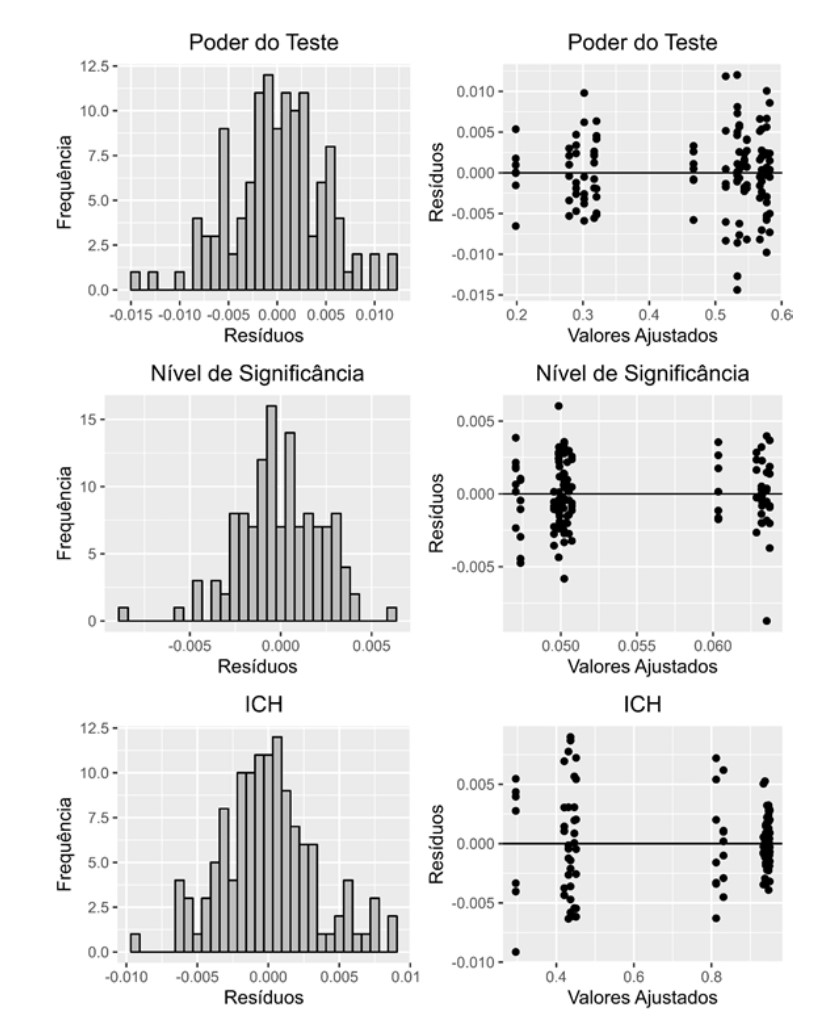
Descargas
Publicado
Cómo citar
Número
Sección
Licencia
Proposta de Política para Periódicos de Acesso Livre
Autores que publicam nesta revista concordam com os seguintes termos:
- Autores mantém os direitos autorais e concedem à revista o direito de primeira publicação, com o trabalho simultaneamente licenciado sob a Licença Creative Commons Attribution que permite o compartilhamento do trabalho com reconhecimento da autoria e publicação inicial nesta revista.
- Autores têm autorização para assumir contratos adicionais separadamente, para distribuição não-exclusiva da versão do trabalho publicada nesta revista (ex.: publicar em repositório institucional ou como capítulo de livro), com reconhecimento de autoria e publicação inicial nesta revista.
- Autores têm permissão e são estimulados a publicar e distribuir seu trabalho online (ex.: em repositórios institucionais ou na sua página pessoal) a qualquer ponto antes ou durante o processo editorial, já que isso pode gerar alterações produtivas, bem como aumentar o impacto e a citação do trabalho publicado (Veja O Efeito do Acesso Livre).