Data transformation under normality and heteroscedasticity
a simulation study
Keywords:
Power of the test, significance level, pressupositionsAbstract
Analysis of Variance (ANOVA) is one of the most used inferential techniques in biological, ecological and agricultural sciences and it depends heavily on the assumption of equal variances along the treatments. Little is known about the properties of the F-test with different transformations under normality and heteroscedasticity. Therefore, this study aims to evaluate the impact of transformation on the power and significance level of F-test under heteroscedasticity. To do that, a simulation study was carried out using 6 transformations, 3 levels of non-constant variances, 7 repetitions and 10.000 simulated data sets. The mean proportions 5-5-5 and 5-5-6 were used to assess the significance level and power, respectively. Bartlett test was carried out on each simulated data set in order to define the Homogenization Capacity Index (HCI) to each transformation. The results were analyzed using ANOVA F-test at the level of 0.05 of the significance and compared using Tukey test after evaluating the presuppositions. Data transformation does not improve the power, significance level or the HCI at any level of heteroscedasticity. Since HCI stays around 40% after transformation, it's possible to have positive results in assumptions tests in spite of the quality of the test. The use of data transformation to handle heteroscedasticity in normal data is not an effective strategy and robust tests should be used.
References
ASLAM, Muhammad. Design of the Bartlett and Hartley tests for homogeneity of variances under
indeterminacy environment. Journal Of Taibah University For Science, [S.L.], v. 14, n. 1, p. 6-10, 10 dez.
Informa UK Limited. http://dx.doi.org/10.1080/16583655.2019.1700675.
BLANCA, María J.; ALARCÓN, Rafael; ARNAU, Jaume. Non-normal data: is anova still a valid option?.
Psicothema, [S.L.], n. 294, p. 552-557, nov. 2017. C.O.P. del Ppdo. de Asturias.
http://dx.doi.org/10.7334/psicothema2016.383.
BLANCA, María J.; ALARCÓN, Rafael; ARNAU, Jaume; BONO, Roser; BENDAYAN, Rebecca. Effect of
variance ratio on ANOVA robustness: might 1.5 be the limit?. Behavior Research Methods, [S.L.], v. 50, n.
, p. 937-962, 22 jun. 2017. Springer Science and Business Media LLC. http://dx.doi.org/10.3758/s13428-
-0918-2.
FERNANDEZ, George C.J.. Residual Analysis and Data Transformations: important tools in statistical
analysis. Hortscience, [S.L.], v. 27, n. 4, p. 297-300, abr. 1992. American Society for Horticultural Science.
http://dx.doi.org/10.21273/hortsci.27.4.297.
KARIYA, Takeaki; KURATA, Hiroshi. Generalized Least Squares. Wiley Series In Probability And
Statistics, [S.L.], p. 500-545, 25 jun. 2004. Wiley. http://dx.doi.org/10.1002/0470866993.
KNIEF, Ulrich; FORSTMEIER, Wolfgang. Violating the normality assumption may be the lesser of two evils.
Behavior Research Methods, [S.L.], v. 53, n. 6, p. 2576-2590, 7 maio 2021. Springer Science and Business
Media LLC. http://dx.doi.org/10.3758/s13428-021-01587-5.
Kozak, M. Analyzing one-way experiments: a piece of cake or a pain in the neck. Sci. Agric, 4, 556–562.
https://www.scielo.br/j/sa/a/6nsk8bJv9zsyWR7SbPfwmQG/?format=pdf.
MAHAPOONYANONT, Natcha; MAHAPOONYANONT, Tharadeth; PENGKAEW, Nussara;
KAMHANGKIT, Rojarek. Power of the test of One-Way Anova after transforming with large sample size
data. Procedia - Social And Behavioral Sciences, [S.L.], v. 9, p. 933-937, 2010. Elsevier BV.
http://dx.doi.org/10.1016/j.sbspro.2010.12.262.
MODER, K. Alternatives to F-test in one way ANOVA in case of heterogeneity of variances (a simulation
study). Psychological Test and Assessment Modeling, 52(4), 343–353, 2010.
MORRIS, Tim P.; WHITE, Ian R.; CROWTHER, Michael J.. Using simulation studies to evaluate statistical
methods. Statistics In Medicine, [S.L.], v. 38, n. 11, p. 2074-2102, 16 jan. 2019. Wiley.
http://dx.doi.org/10.1002/sim.8086.
OSBORNE, Jason. Notes on the use of data transformations. University Of Massachusetts Amherst, [S.L.],
p. 37-45, 2002. University of Massachusetts Amherst. http://dx.doi.org/10.7275/4VNG-5608.
PEK, J.; WONG, O.; WONG, A. C.. Data Transformations for Inference with Linear Regression: clarifications
and recommendations. University Of Massachusetts Amherst, [S.L.], p. 22-35, 2017. University of
Massachusetts Amherst. http://dx.doi.org/10.7275/2W3N-0F07.
SNEDECOR, G. W.. Statistical methods applied to experiments in agriculture and biology, 1st–5th editions.
Ames, IA: Collegiate Press, 1956.
STANTON, Maureen L.; THIEDE, Denise A.. Statistical convenience vs biological insight: consequences of
data transformation for the analysis of fitness variation in heterogeneous environments. New Phytologist,
[S.L.], v. 166, n. 1, p. 319-338, 12 jan. 2005. Wiley. http://dx.doi.org/10.1111/j.1469-8137.2004.01311.x.
ST‐PIERRE, Anne P.; SHIKON, Violaine; SCHNEIDER, David C.. Count data in biology—Data
transformation or model reformation? Ecology And Evolution, [S.L.], v. 8, n. 6, p. 3077-3085, 16 fev. 2018.
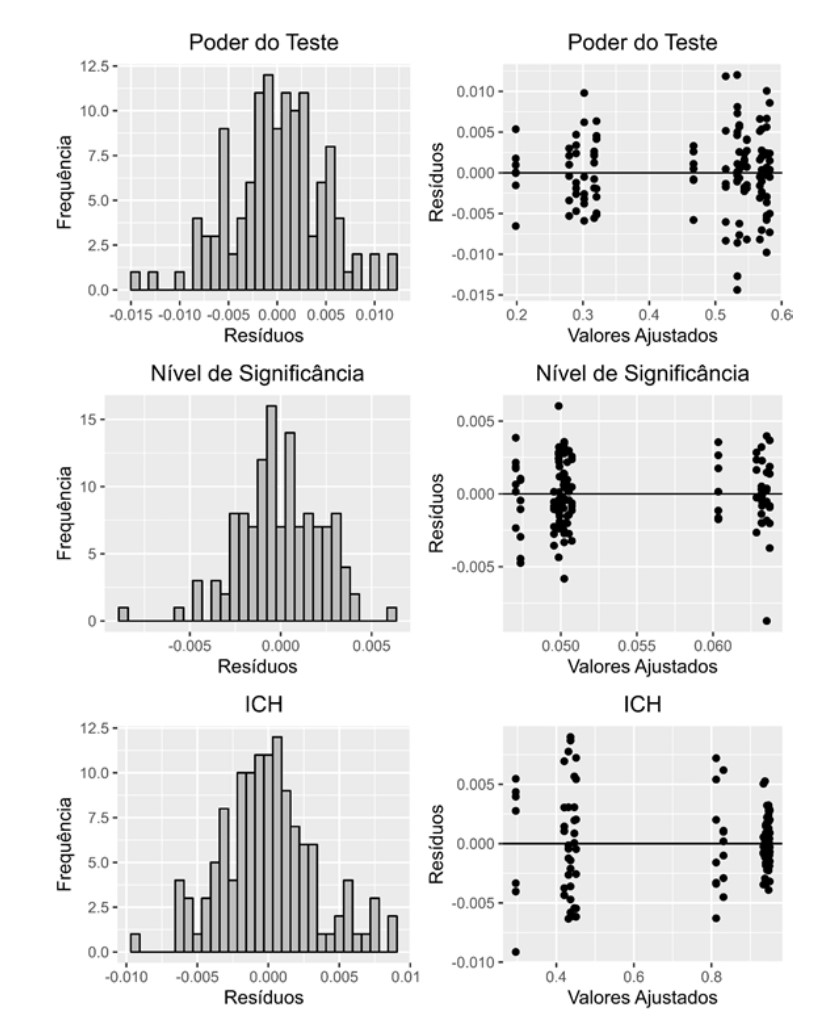
Downloads
Published
How to Cite
Issue
Section
License
Proposta de Política para Periódicos de Acesso Livre
Autores que publicam nesta revista concordam com os seguintes termos:
- Autores mantém os direitos autorais e concedem à revista o direito de primeira publicação, com o trabalho simultaneamente licenciado sob a Licença Creative Commons Attribution que permite o compartilhamento do trabalho com reconhecimento da autoria e publicação inicial nesta revista.
- Autores têm autorização para assumir contratos adicionais separadamente, para distribuição não-exclusiva da versão do trabalho publicada nesta revista (ex.: publicar em repositório institucional ou como capítulo de livro), com reconhecimento de autoria e publicação inicial nesta revista.
- Autores têm permissão e são estimulados a publicar e distribuir seu trabalho online (ex.: em repositórios institucionais ou na sua página pessoal) a qualquer ponto antes ou durante o processo editorial, já que isso pode gerar alterações produtivas, bem como aumentar o impacto e a citação do trabalho publicado (Veja O Efeito do Acesso Livre).