Modelagem de séries temporais de contagem
um estudo comparativo de caso
Palavras-chave:
Modelo Observation-driven, GAM-ARMA, NGSSEML, Modelo Parameter-driven, dados de contagemResumo
Esse artigo apresenta uma aplicação a dados de contagem, onde os modelos observation-driven e parameter-driven são comparados. Com esse propósito, os modelos Generalizado Aditivo Autorregressivo Média Móvel (GAM-ARMA) e Espaço de Estados Não-Gaussiano com Verossimilhança Marginal Exata (NGSSEML) são utilizados. Parâmetros dos modelos são estimados utilizando o método de máxima verossimilhança. A capacidade do procedimento de modelar e prever dados reais é apresentada para o número de casos de doença obstrutiva crônica (COPD).
Referências
O. Y. E. Albarracin, A. P. Alencar, and L. L. Ho. Generalized autoregressive and moving average
models: multicollinearity, interpretation and a new modified model. Journal of Statistical
Computation and Simulation, 89(10):1819–1840, 2019.
M. A. Benjamin, R. A. Rigby, and D. M. Stasinopoulos. Generalized autoregressive moving
average models. Journal of the American Statistical association, 98(461):214–223, 2003.
A. J. A. Camara, G. C. Franco, V. A. Reisen, and P. Bondon. Generalized additive model for
count time series: An application to quantify the impact of air pollutants on human health.
Pesquisa Operacional, 41, 2021.
D. R. Cox. Statistical analysis of time series: Some recent developments. Scandinavian Journal
of Statistics, pages 93–115, 1981.
R. A. Davis and H. Liu. Theory and inference for a class of observation-driven models with
application to time series of counts. arXiv preprint arXiv:1204.3915, 2012.
R. A. Davis and R. Wu. A negative binomial model for time series of counts. Biometrika, 96
(3):735–749, 2009.
R. A. Davis, W. T. Dunsmuir, and Y. Wang. Modeling time series of count data. Statistics
textbooks and monographs, 158:63–114, 1999.
R. A. Davis, W. T. Dunsmuir, and Y. Wang. On autocorrelation in a poisson regression model.
Biometrika, 87(3):491–505, 2000.
R. A. Davis, W. T. Dunsmuir, and S. B. Streett. Observation-driven models for poisson counts.
Biometrika, 90(4):777–790, 2003.
C. De Boor. A practical guide to splines, volume 27. springer-verlag New York, 1978.
G. de Oliveira Maia, W. Barreto-Souza, F. de Souza Bastos, and H. Ombao. Semiparametric
time series models driven by latent factor. International Journal of Forecasting, 37(4):1463–
, 2021.
W. T. Dunsmuir and D. J. Scott. The glarma package for observation-driven time series regres sion of counts. Journal of Statistical Software, 67:1–36, 2015.
P. H. C. Eilers and B. D. Marx. Flexible smoothing with B-splines and penal ties. Statistical Science, 11(2):89 – 121, 1996. doi: 10.1214/ss/1038425655. URL https://doi.org/10.1214/ss/1038425655.
G. C. Franco, H. S. Migon, and M. O. Prates. A monte carlo study and data analysis for
time series of counts. Relat´orio T´ecnico Relat´orio T´ecnico RTP-01/2015, Belo Horizonte:
Departamento de Estatıstica-UFMG, 2015.
J. H. Friedman and B. W. Silverman. Flexible parsimonious smoothing and ad ditive modeling. Technometrics, 31(1):3–21, 1989. ISSN 00401706. URL
http://www.jstor.org/stable/1270359.
D. Gamerman, T. R. dos Santos, and G. C. Franco. A non-gaussian family of state-space models
with exact marginal likelihood. Journal of Time Series Analysis, 34(6):625–645, 2013.
P. J. Green and B. W. Silverman. Nonparametric regression and generalized linear models: a
roughness penalty approach. Crc Press, 1994.
F. E. Harrel. Bioestatistical modeling. Nashvile TN USA, 2004.
T. Hastie and R. Tibshirani. Generalized Additive Models. Chapman & Hall/CRC Monographs
on Statistics & Applied Probability. Taylor & Francis, 1990. ISBN 9780412343902. URL
https://books.google.com.br/books?id=qa29r1Ze1coC.
R. C. Jung and A. R. Tremayne. Useful models for time series of counts or simply wrong ones?
AStA Advances in Statistical Analysis, 95:59–91, 2011.
R. C. Jung, M. Kukuk, and R. Liesenfeld. Time series of count data: modeling, estimation and
diagnostics. Computational Statistics & Data Analysis, 51(4):2350–2364, 2006.
C. Kooperberg and C. J. Stone. A study of logspline density estimation. Computational Statistics
& Data Analysis, 12(3):327–347, 1991.
M. Melo and A. Alencar. Conway–maxwell–poisson autoregressive moving average model for
equidispersed, underdispersed, and overdispersed count data. Journal of Time Series Analysis,
(6):830–857, 2020.
A. V. Rocha and F. Cribari-Neto. Beta autoregressive moving average models. Test, 18:529–545,
S. L. Zeger. A regression model for time series of counts. Biometrika, 75(4):621–629, 1988.
S. L. Zeger and B. Qaqish. Markov regression models for time series: a quasi-likelihood approach.
Biometrics, pages 1019–1031, 1988.
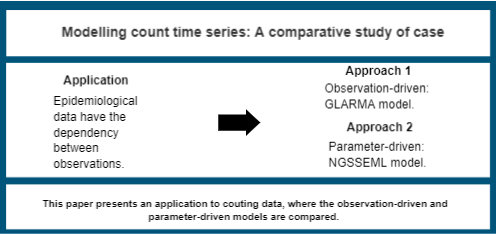
Downloads
Publicado
Versões
- 11-04-2024 (2)
- 20-03-2024 (1)
Como Citar
Edição
Seção
Licença
Proposta de Política para Periódicos de Acesso Livre
Autores que publicam nesta revista concordam com os seguintes termos:
- Autores mantém os direitos autorais e concedem à revista o direito de primeira publicação, com o trabalho simultaneamente licenciado sob a Licença Creative Commons Attribution que permite o compartilhamento do trabalho com reconhecimento da autoria e publicação inicial nesta revista.
- Autores têm autorização para assumir contratos adicionais separadamente, para distribuição não-exclusiva da versão do trabalho publicada nesta revista (ex.: publicar em repositório institucional ou como capítulo de livro), com reconhecimento de autoria e publicação inicial nesta revista.
- Autores têm permissão e são estimulados a publicar e distribuir seu trabalho online (ex.: em repositórios institucionais ou na sua página pessoal) a qualquer ponto antes ou durante o processo editorial, já que isso pode gerar alterações produtivas, bem como aumentar o impacto e a citação do trabalho publicado (Veja O Efeito do Acesso Livre).