Efeito de encolhimento na análise bayesiana do modelo GGE utilizando priori de máxima entropia
Palavras-chave:
Modelo GGE, bayesiana, máxima entropiaResumo
Neste trabalho, utilizou-se o princípio de máxima entropia para atribuir informação a priori às componentes de variância dos valores singulares no modelo de efeitos principais de genótipos mais interação genótipo×ambientes (GGE). O método foi exemplificado a partir de um conjunto de dados simulados. Os resultados mostraram que o modelo GGE com priori de máxima entropia (BGGEE) produz efeito de encolhimento nas estimativas dos valores singulares, quando comparado com a análise GGE frequentista ou com a versão bayesiana GGE que utiliza priori não informativa (referida por BGGE). O BGGEE mostrou maior parcimônia, estimando os valores singulares com maior contribuição para o efeito da interação e encolhendo as estimativas de valores singulares associados a maiores dimensões a zero. Assim, o BGGEE capturou mais padrão e descartou mais ruído do que a versão Bayesiana típica. Ao utilizar priori de máxima entropia, constatou-se que o modelo completo e aquele com apenas dois termos bilineares são quase indistinguíveis. Isso sinaliza que a seleção do modelo no ajuste BGGEE não seria uma etapa necessária. O método também evita problemas de amostragem observados quando a priori de Jeffreys é utilizada, resultando em distribuições marginais a posteriori próprias e uni modais.
Referências
BURGUEÑO, J. et al. Using factor analytic models for joining environments and genotypes without crossover genotype _ environment interaction. Crop Science, v. 48, n. 4, p. 1291-1305, 2008.
CHEN, M. H.; SHAO, Q. M. Monte Carlo estimation of Bayesian credible and HPD intervals. Journal of Computational and Graphical Statistics, v. 8, n. 1, p. 69-92, 1999.
CORNELIUS, P.; SEYEDSADR, M. Estimation of general linear-bilinear models for two-way tables. Journal of Statistical Computation and Simulation, v. 58, n. 4, p. 287-322, 1997.
CORNELIUS, P.; CROSSA, J.; SEYEDSADR, M. Statistical tests and estimators of multiplicative models for genotype-by-environment interaction. In:-------- . Genotype-byenvironment interaction. Boca Raton: CRC, 1996. p. 199-234.
CORNELIUS, P. L.; CROSSA, J. Prediction assessment of shrinkage estimators of multiplicative models for multi-environment cultivar trials. Crop Science, v. 39, n. 4, p. 998-1009, 1999.
CORNELIUS, P. Statistical tests and retention of terms in the additive main effects and multiplicative interaction model for cultivar trials. Crop Science, v. 33, n. 6, p. 1186-1193, 1993.
CROSSA, J.; YANG, R.-C.; CORNELIUS, P. L. Studying crossover genotype environment interaction using linear-bilinear models and mixed models. Journal of Agricultural, Biological, and Environmental Statistics, v. 9, n. 3, p. 362-380, 2004.
CROSSA, J. et al. Bayesian estimation of the additive main effects and multiplicative interaction model. Crop Science, v. 51, n. 4, p. 1458-1469, 2011.
CRUZ, C.; REGAZZI, A.; CARNEIRO, P. C. Modelos biométricos aplicados ao melhoramento genético. 4. ed. Viçosa: UFV, 2012. 514 p.
CROSSA, J.; CORNELIUS, P. L. Sites regression and shifted multiplicative model clustering of cultivar trial sites under heterogeneity of error variances. Crop Science, v. 37, n. 2, p. 406-415, 1997.
DENIS, J.-B.; GOWER, J. C. Asymptotic covariances for the parameters of biadditive models. Utilitas Mathematica, v. 46, p. 193-205, 1994.
HEIDELBERGER, P.; WELCH, P. D. Simulation run length control in the presence of an initial transient. Operations Research, v. 31, n. 6, p. 1109-1144, 1983.
HU, Z.; YANG, R. Improved statistical inference for graphical description and
interpretation of genotype times environment interaction. Crop Science, v. 53, n. 6, p.2400-2410, 2013.
JARQUÍN, D. et al. A hierarchical Bayesian estimation model for multi-environment plant breeding trials in successive years. Crop Science, v. 56, n.5, p. 2260-2276, 2016.
JAYNES, E. T. Information theory and statistical mechanics. Physical Review, APS, v. 106, n. 4, p. 620-630, 1957.
JAYNES, E. T. Probability theory: The logic of science. New York: Cambridge University Press, 2003. 757 p.
JEFFREYS, H. Theory of Probability. 3. ed. Oxford: Oxford Classic Texts in the Physical Sciences, 1961. 459 p.
LIU, G. Bayesian computations for general linear-bilinear models. 2001. 169 p. Thesis (Doctor of Philosophy) - University of Kentucky, Lexington, 2001.
OLIVEIRA, L. A. et al. Credible intervals for scores in the AMMI with random effects for genotype. Crop Science, v. 55, n. 2, p. 465-476, 2015.
OLIVEIRA, L. A. de et al. Bayesian GGE biplot models applied to maize multienvironments trials. Genetics and Molecular Research, v. 15, n. 2, p. 1-21, 2016.
PEREZ-ELIZALDE, S.; JARQUIN, D.; CROSSA, J. A general Bayesian estimation method of linear–bilinear models applied to plant breeding trials with genotype×environment interaction. Journal of Agricultural, Biological, and Environmental Statistics, v. 17, n. 1, p. 15-37, 2012.
R CORE TEAM. R: A language and environment for statistical computing. R Foundation for Statistical Computing, Vienna, Austria. 2019. URL: http://www.R-project.org/
RAFTERY, A. E. et al. Estimating the integrated likelihood via posterior simulation using the harmonic mean identity. In: ____. Bayesian Statistics. [S.l.], 2007. v. 8, p. 1-45.
RAFTERY, A. E.; LEWIS, S. M. [Practical Markov Chain Monte Carlo]: comment: one long run with diagnostics: implementation strategies for Markov Chain Monte Carlo. Statistical Science, v. 7, n. 4, p. 493-497, 1992.
ROMÃO, R. F. et al. Predictive ability of AMMI and factorial analytical models in the study of unbalanced multi-environment data. Genetics and Molecular Research, v. 18, n. 3, p. 1-20, 2019.
SILVA, C. P. et al. A Bayesian Shrinkage approach for AMMI Models. PLoS One, v.10, n. 7, p. e0131414, 2015.
SILVA, C. P. et al. Heterogeneity of variances in the Bayesian AMMI model for multienvironment trial studies. Crop Science, v. 59, n. 6, p. 2455-2472, 2019.
TER BRAAK, C.J.F; BOER, M.P.; BINK, M. C.A.M. Extending Xu's Bayesian model for estimating polygenic effects using markers of the entire genome. Genetics, v. 170, n. 3, p. 1435-1438, 2005.
VIELE, K.; SRINIVASAN, C. Parsimonious estimation of multiplicative interaction in analysis of variance using Kullback-Leibler information. Journal of Statistical Planning and Inference, v. 84, n. 1-2, p. 201-219, 2000.
YAN, W.; GLOVER, K. D.; KANG, M. S. Comment on “biplot analysis of genotype _environment interaction: proceed with caution” by R.-C. Yang, J. Crossa, P.L. Cornelius, and J. Burgueño in 2009, v.49, n.5: 1564-1576. Crop Science, v. 50, n. 4, p. 1121-1123, 2010.
YANG, R.-C. et al. Biplot analysis of genotype _ environment interaction: proceed with caution. Crop Science, v. 49, n. 5, p. 1564-1576, 2009.
YAN, W. Crop Variety Trials: Data Management and Analysis. New York: John Wiley & Sons, 2014. 361 p.
YAN, W et al. Cultivar evaluation and mega-environment investigation based on the GGE biplot. Crop Science, v. 40, n. 3, p. 597-605, 2000.
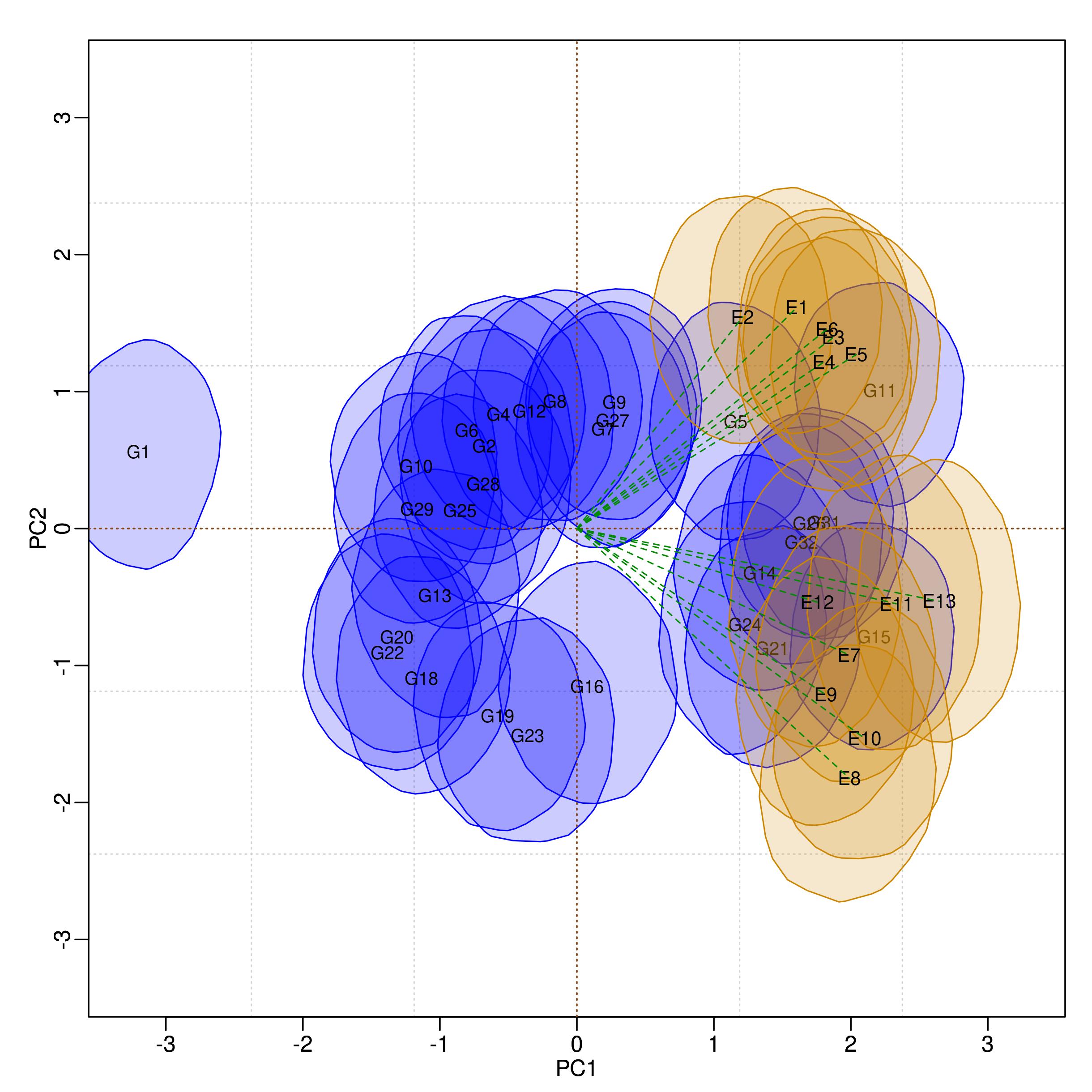
Downloads
Publicado
Como Citar
Edição
Seção
Licença
Proposta de Política para Periódicos de Acesso Livre
Autores que publicam nesta revista concordam com os seguintes termos:
- Autores mantém os direitos autorais e concedem à revista o direito de primeira publicação, com o trabalho simultaneamente licenciado sob a Licença Creative Commons Attribution que permite o compartilhamento do trabalho com reconhecimento da autoria e publicação inicial nesta revista.
- Autores têm autorização para assumir contratos adicionais separadamente, para distribuição não-exclusiva da versão do trabalho publicada nesta revista (ex.: publicar em repositório institucional ou como capítulo de livro), com reconhecimento de autoria e publicação inicial nesta revista.
- Autores têm permissão e são estimulados a publicar e distribuir seu trabalho online (ex.: em repositórios institucionais ou na sua página pessoal) a qualquer ponto antes ou durante o processo editorial, já que isso pode gerar alterações produtivas, bem como aumentar o impacto e a citação do trabalho publicado (Veja O Efeito do Acesso Livre).