Modeling count time series
a comparative case study
Palabras clave:
Observation-driven model, Parameter-driven model, GAM-ARMA, NGSSEML, counting dataResumen
This paper presents an application for counting data, where the observation-driven and parameter-driven models are compared. To this purpose, the Generalized Additive Autoregressive Moving Average (GAM-ARMA) and Non-Gaussian State Space with Exact Marginal Likelihood (NGSSEML) models are used. Model parameters are estimated using the maximum likelihood method. The ability of the procedure to model and forecast real data is presented for the number of chronic obstructive disease (COPD) cases.
Citas
O. Y. E. Albarracin, A. P. Alencar, and L. L. Ho. Generalized autoregressive and moving average
models: multicollinearity, interpretation and a new modified model. Journal of Statistical
Computation and Simulation, 89(10):1819–1840, 2019.
M. A. Benjamin, R. A. Rigby, and D. M. Stasinopoulos. Generalized autoregressive moving
average models. Journal of the American Statistical association, 98(461):214–223, 2003.
A. J. A. Camara, G. C. Franco, V. A. Reisen, and P. Bondon. Generalized additive model for
count time series: An application to quantify the impact of air pollutants on human health.
Pesquisa Operacional, 41, 2021.
D. R. Cox. Statistical analysis of time series: Some recent developments. Scandinavian Journal
of Statistics, pages 93–115, 1981.
R. A. Davis and H. Liu. Theory and inference for a class of observation-driven models with
application to time series of counts. arXiv preprint arXiv:1204.3915, 2012.
R. A. Davis and R. Wu. A negative binomial model for time series of counts. Biometrika, 96
(3):735–749, 2009.
R. A. Davis, W. T. Dunsmuir, and Y. Wang. Modeling time series of count data. Statistics
textbooks and monographs, 158:63–114, 1999.
R. A. Davis, W. T. Dunsmuir, and Y. Wang. On autocorrelation in a poisson regression model.
Biometrika, 87(3):491–505, 2000.
R. A. Davis, W. T. Dunsmuir, and S. B. Streett. Observation-driven models for poisson counts.
Biometrika, 90(4):777–790, 2003.
C. De Boor. A practical guide to splines, volume 27. springer-verlag New York, 1978.
G. de Oliveira Maia, W. Barreto-Souza, F. de Souza Bastos, and H. Ombao. Semiparametric
time series models driven by latent factor. International Journal of Forecasting, 37(4):1463–
, 2021.
W. T. Dunsmuir and D. J. Scott. The glarma package for observation-driven time series regres sion of counts. Journal of Statistical Software, 67:1–36, 2015.
P. H. C. Eilers and B. D. Marx. Flexible smoothing with B-splines and penal ties. Statistical Science, 11(2):89 – 121, 1996. doi: 10.1214/ss/1038425655. URL https://doi.org/10.1214/ss/1038425655.
G. C. Franco, H. S. Migon, and M. O. Prates. A monte carlo study and data analysis for
time series of counts. Relat´orio T´ecnico Relat´orio T´ecnico RTP-01/2015, Belo Horizonte:
Departamento de Estatıstica-UFMG, 2015.
J. H. Friedman and B. W. Silverman. Flexible parsimonious smoothing and ad ditive modeling. Technometrics, 31(1):3–21, 1989. ISSN 00401706. URL
http://www.jstor.org/stable/1270359.
D. Gamerman, T. R. dos Santos, and G. C. Franco. A non-gaussian family of state-space models
with exact marginal likelihood. Journal of Time Series Analysis, 34(6):625–645, 2013.
P. J. Green and B. W. Silverman. Nonparametric regression and generalized linear models: a
roughness penalty approach. Crc Press, 1994.
F. E. Harrel. Bioestatistical modeling. Nashvile TN USA, 2004.
T. Hastie and R. Tibshirani. Generalized Additive Models. Chapman & Hall/CRC Monographs
on Statistics & Applied Probability. Taylor & Francis, 1990. ISBN 9780412343902. URL
https://books.google.com.br/books?id=qa29r1Ze1coC.
R. C. Jung and A. R. Tremayne. Useful models for time series of counts or simply wrong ones?
AStA Advances in Statistical Analysis, 95:59–91, 2011.
R. C. Jung, M. Kukuk, and R. Liesenfeld. Time series of count data: modeling, estimation and
diagnostics. Computational Statistics & Data Analysis, 51(4):2350–2364, 2006.
C. Kooperberg and C. J. Stone. A study of logspline density estimation. Computational Statistics
& Data Analysis, 12(3):327–347, 1991.
M. Melo and A. Alencar. Conway–maxwell–poisson autoregressive moving average model for
equidispersed, underdispersed, and overdispersed count data. Journal of Time Series Analysis,
(6):830–857, 2020.
A. V. Rocha and F. Cribari-Neto. Beta autoregressive moving average models. Test, 18:529–545,
S. L. Zeger. A regression model for time series of counts. Biometrika, 75(4):621–629, 1988.
S. L. Zeger and B. Qaqish. Markov regression models for time series: a quasi-likelihood approach.
Biometrics, pages 1019–1031, 1988.
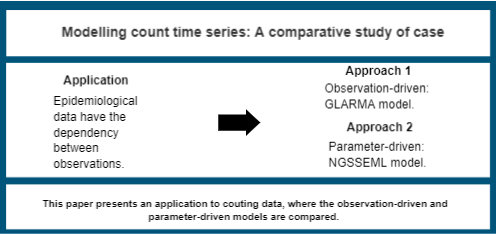
Descargas
Publicado
Versiones
- 11-04-2024 (2)
- 20-03-2024 (1)
Cómo citar
Número
Sección
Licencia
Proposta de Política para Periódicos de Acesso Livre
Autores que publicam nesta revista concordam com os seguintes termos:
- Autores mantém os direitos autorais e concedem à revista o direito de primeira publicação, com o trabalho simultaneamente licenciado sob a Licença Creative Commons Attribution que permite o compartilhamento do trabalho com reconhecimento da autoria e publicação inicial nesta revista.
- Autores têm autorização para assumir contratos adicionais separadamente, para distribuição não-exclusiva da versão do trabalho publicada nesta revista (ex.: publicar em repositório institucional ou como capítulo de livro), com reconhecimento de autoria e publicação inicial nesta revista.
- Autores têm permissão e são estimulados a publicar e distribuir seu trabalho online (ex.: em repositórios institucionais ou na sua página pessoal) a qualquer ponto antes ou durante o processo editorial, já que isso pode gerar alterações produtivas, bem como aumentar o impacto e a citação do trabalho publicado (Veja O Efeito do Acesso Livre).