GAMLSS Models: Application in cases of Severe Acute Respiratory Syndrome with emphasis on Influenza and other causes
Keywords:
Statistical Modeling, GAMLSS Regression, SRAG, InfluenzaAbstract
One of the major causes of Severe Acute Respiratory Syndrome (SARS) is Influenza, a respiratory disease caused by several strains of the virus. The global spread of Influenza has represented a significant challenge since 2009. In this context, this research proposes to analyze SARS with an emphasis on Influenza and other causes (viruses and other etiological agents), in order to investigate and understand the relationship between predictor variables (comorbidities) and the specific response variable (evolution) in the cases registered in Brazil. From the analysis of the results found, referring to the period from 2020 to August 2022, it was noted that the data indicated an unsatisfactory fit to the Multiple Linear Regression model and the Generalized Linear Models, which motivated the use of Generalized Additive Models for Location, Scale and Shape. To analyze these data, we used classical probability distributions in the context of this modeling: binomial, negative binomial, geometric and Poisson. Of these distributions worked, the binomial distribution proved to be effective, allowing us to map the adequacy of this modeling. Our training and test sets were performed in order to verify possible overfitting in the obtained model. This approach provided a deeper understanding of the data set and the relationships between the studied variations. Asthmatic patients demonstrated a strong and significant association with the mortality rate, while those with chronic liver conditions had a lower risk of death. These results provide important strategies for intervention and care of populations affected by these health conditions.
References
AKANTZILIOTOU, C., RIGBY, R. A., & STASINOPOULOS, D. M. (2002). The R Implementation of Generalized Additive Models for Location, Scale and Shape. In M. Stasinopoulos & G. Touloumi (Eds.), Statistical Modelling in Society: Proceedings of the 17th International Workshop on Statistical Modelling (pp. 75–83). Chania, Greece.
BRASIL. (2009). Estratégia Nacional de Vacinação contra o Vírus Influenza Pandêmico (H1N1) 2009. Ministério da Saúde. Brasília, DF. Recuperado de https://bvsms.saude.gov.br/bvs/publicacoes/estrategia_nacional_vacinacao_influenza.pdf
BRASIL. (2022). Dicionário de dados. Ministério da Saúde. Secretária de Vigilância em Saúde. Sistema de Informação de Vigilância Epidemiológica da Gripe. Recuperado de https://s3.sa-east-1.amazonaws.com/ckan.saude.gov.br/SRAG/pdfs/dicionario_de_dados_srag_hosp_17_02_2022.pdf
BRASIL. (2020). Protocolo de Manejo Clínico. Ministério da Saúde. Brasília, DF. Recuperado de https://www.saude.ms.gov.br/wp-content/uploads/2020/03/Protocolo-Manejo-Clinico_APS_versao04.pdf
DATASUS. (2022). Ministério da Saúde. SRAG - Banco de Dados de Síndrome Respiratória Aguda Grave - incluindo dados da COVID-19. OpenDataSUS. Recuperado de https://opendatasus.saude.gov.br/dataset/srag-2021-e-2022
FREITAG, V. L., ANTONIO, M. G. D., LOUREIRO, L. H., & PEREIRA, R. M. S. (2021). COVID 19 e a propagação de fake news sobre a contaminação pelo dióxido de carbono com o uso de máscaras faciais: Um estudo de reflexão. Research, Society and Development, 10(10), e104101018696. https://doi.org/10.33448/rsd-v10i10.18696
GONZALEZ, TEOFILO; SAHNI, SARTAJ; FRANTA & WILLIAM R. (1977). An efficient algorithm for the Kolmogorov-Smirnov and Lilliefors tests. ACM Transactions on Mathematical Software (TOMS), v. 3, n. 1, p. 60-64.
HASTIE, T. J., & TIBSHIRANI, R. J. (1990). Generalized Additive Models. Chapman and Hall.
LI, S., & YANG, B. (2008). Region-based multi-focus image fusion. https://doi.org/10.1016/B978-0-12-372529-5.00009-3
MAGALHÃES, M. N. (2015). Probabilidade e Variáveis Aleatórias. EDUSP.
NELDER, J. A., & WEDDERBURN, R. W. M. (1972). Generalized linear models. Journal of the Royal Statistical Society, A, 135, 370–384.
RIBEIRO, M. L. F., CORDEIRO, N. M., & ALVES, D. A. N. DA S. (2022). Aplicação de modelagem preditiva via árvore de decisão em casos de Síndrome Respiratória Aguda Grave (SRAG), com ênfase na Doença por Corona Vírus 2019 (COVID-19) no Brasil para o período de 2020 a 2022. Research, Society and Development, 11(15), e01111536173. https://doi.org/10.33448/rsd-v11i15.36173
RIGBY, R. A., & STASINOPULOUS, D. M. (2005). Generalized Additive Models for Location, Scale and Shape. Applied Statistics, 54, 507–554.
RIGBY, R. A., & STASINOPULOUS, D. M. (2001). The GAMLSS project: a Flexible Approach to Statistical Modelling. In B. Klein & L. Korsholm (Eds.), New Trends in Statistical Modelling: Proceedings of the 16th International Workshop on Statistical Modelling (pp. 249–256). Odense, Denmark.
SAMPERI, R. H., COLLADO, C. F., & LUCIO, M. DEL P. B. (2013). Metodologia Científica. AMGH Editora.
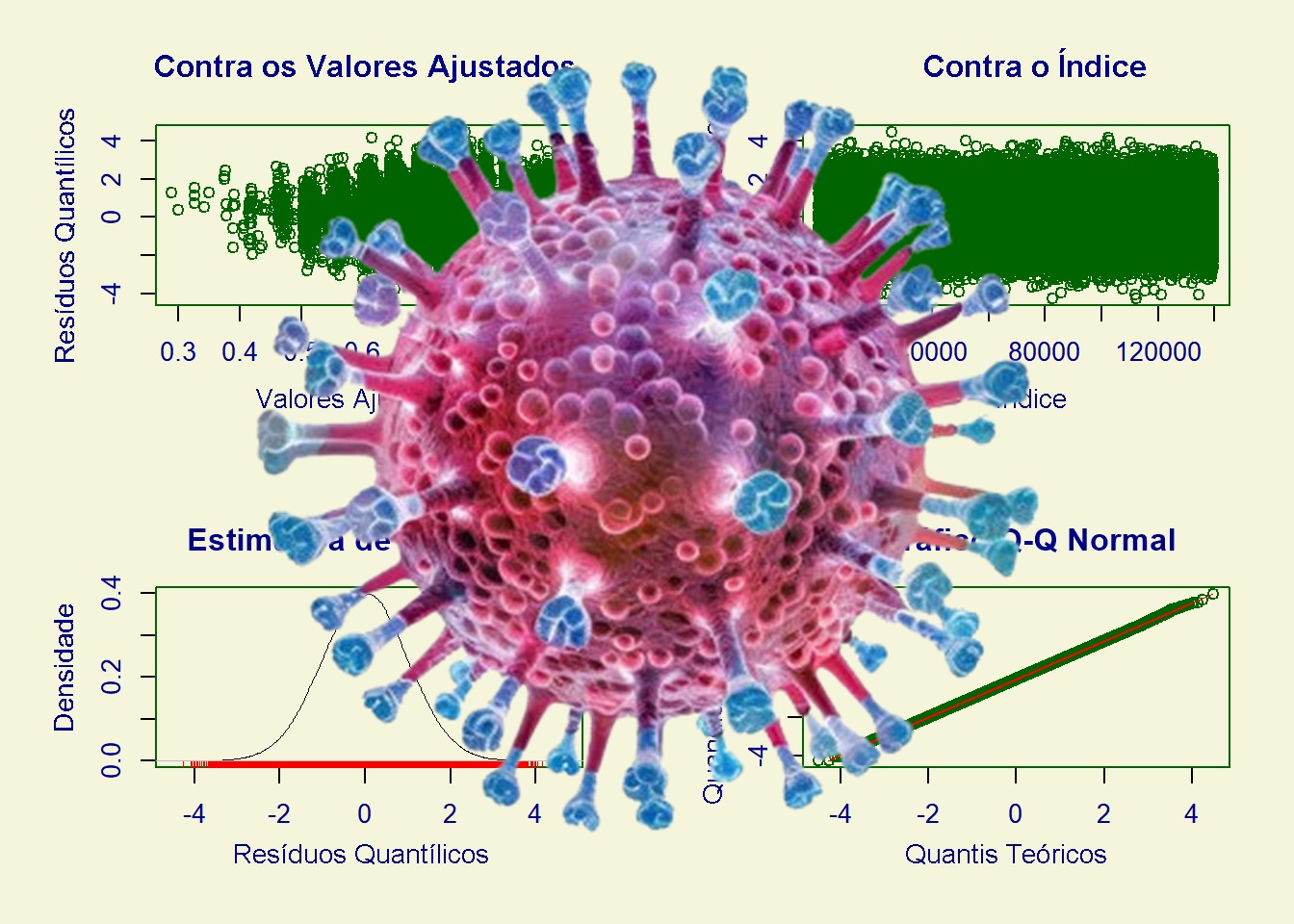
Downloads
Published
How to Cite
Issue
Section
License
Proposta de Política para Periódicos de Acesso Livre
Autores que publicam nesta revista concordam com os seguintes termos:
- Autores mantém os direitos autorais e concedem à revista o direito de primeira publicação, com o trabalho simultaneamente licenciado sob a Licença Creative Commons Attribution que permite o compartilhamento do trabalho com reconhecimento da autoria e publicação inicial nesta revista.
- Autores têm autorização para assumir contratos adicionais separadamente, para distribuição não-exclusiva da versão do trabalho publicada nesta revista (ex.: publicar em repositório institucional ou como capítulo de livro), com reconhecimento de autoria e publicação inicial nesta revista.
- Autores têm permissão e são estimulados a publicar e distribuir seu trabalho online (ex.: em repositórios institucionais ou na sua página pessoal) a qualquer ponto antes ou durante o processo editorial, já que isso pode gerar alterações produtivas, bem como aumentar o impacto e a citação do trabalho publicado (Veja O Efeito do Acesso Livre).