Clustering Methods for Textual Data Analysis of Strategic Planning Indicators of the State of Mato Grosso
Keywords:
Text Clustering, Exploratory Analysis of Textual Data, Public PolicyAbstract
Indicators play an essential role in effective resource management and monitoring public policies. In Mato Grosso, the Strategic Planning Management Program aims to improve public governance by adopting standardized indicators. This study explores the use of text mining and clustering methods to analyze 160 indicators distributed across ten strategic dimensions: Learning and Knowledge; Economic development; Education; Sports, Culture, and Leisure; Organizational structure; Supervisor; Infrastructure, Basic Sanitation, and Environment; Society Satisfaction; Health and Social Vulnerability. Using Ward's method for grouping and the cosine distance metric, the indicators were grouped into two groups formed by textual characteristics. The indicators of the Education dimension were mainly located in the first group, while the other indicators were aggregated in the second group. The results of this work are useful for public managers in making strategic decisions and promoting improvements in municipal public policies.
References
BRASIL. Plano Nacional de Educação (PNE). Lei no 13.005, de 25 de junho de 2014. Brasília: Ministério da Educação (MEC), 2014.
BRASIL. MINISTÉRIO DA SAÚDE. SECRETARIA DE VIGILÂNCIA EM SAÚDE. Diagnóstico Rápido nos municípios para vigilância entomológica do Aedes Aegypti no Brasil – LIRAa: metodologia para avaliação dos índices de Breteau e Predial. Diretoria Técnica de Gestão. Brasília: Ministério da Saúde, 2005. P. 60. (Série A. Normas e Manuais Técnicos). p. 19.
CALIŃSKI, T.; HARABASZ, J. A dendrite method for cluster analysis. Communications in Statistics, Taylor & Francis, v. 3, n. 1, p. 1–27, 1974. DOI: 10.1080/03610927408827101. Disponível em: https://www.tandfonline.com/doi/abs/10.1080/03610927408827101.
FELDMAN, R.; SANGER, J. The Text Mining Handbook: Advanced Approaches in Analyzing Unstructured Data. [S.l.]: Cambridge University Press, 2007.
JANNUZZI, P. M. A importância da informação estatística para as políticas sociais no Brasil: breve reflexão sobre a experiência do passado para considerar no presente. Revista Brasileira de Estudos de População, São Paulo, v. 35, n. 1, e0055, jan. 2018.
KAPLAN, R.; NORTON, D. The Balanced Scorecard—Measures That Drive Performance. Harvard Business Review, n. 1, p. 71–79, jan. 1992. jan-fev.
MANNING, C. D.; RAGHAVAN, P.; SCHÜTZE, H. Introduction to Information Retrieval. [S.l.]: Cambridge University Press, 2008.
MARSHALL, G.; MEIER, K. J. The Political Economy of Local Government. In: FERLIES, E.; LYNN, L. E.; POLLITT, C. (Ed.). The Oxford Handbook of Public Management. Oxford: Oxford University Press, 2004. P. 205–228.
MATO GROSSO. TRIBUNAL DE CONTAS DO ESTADO. Resolução Normativa no 14/2022 de 28 de junho de 2022: Dispõe sobre a instituição do Programa de Apoio à Gestão do Planejamento Estratégico dos Municípios, denominado GPE, no âmbito do Tribunal de Contas do Estado de Mato Grosso. Cuiabá: [s.n.], jun. 2022.
MINER, G. et al. Practical Text Mining and Statistical Analysis for Non-structured Text Data Applications. [S.l.]: Elsevier, 2012.
MORITA, L. H. M.; CRUZ, R. C.; OLIVEIRA, A. C. S. de. Text Mining Methods for Unifying Strategic Planning Indicators in the Municipalities of Mato Grosso. Sigmae, v. 12, n. 3, p. 39–50, dez. 2023. Disponível em: https://publicacoes.unifal-
mg.edu.br/revistas/index.php/sigmae/article/view/2219.
MURTAGH, F.; LEGENDRE, P. Ward’s hierarchical agglomerative clustering method: which algorithms implement Ward’s criterion? Journal of Classification, v. 31, p. 274–295, 2014. DOI: 10.1007/s00357-014-9161-z.
OPENAI. Embeddings API. [S.l.: s.n.], 2024. Retrieved from https://www.openai.com/embeddings-api.
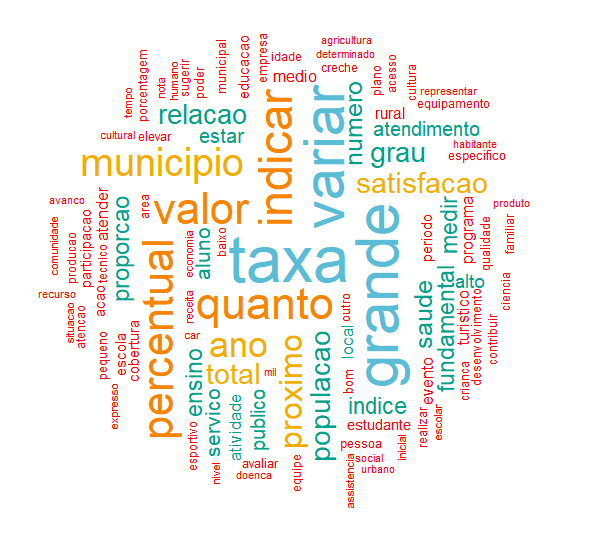
Downloads
Published
How to Cite
Issue
Section
License
Proposta de Política para Periódicos de Acesso Livre
Autores que publicam nesta revista concordam com os seguintes termos:
- Autores mantém os direitos autorais e concedem à revista o direito de primeira publicação, com o trabalho simultaneamente licenciado sob a Licença Creative Commons Attribution que permite o compartilhamento do trabalho com reconhecimento da autoria e publicação inicial nesta revista.
- Autores têm autorização para assumir contratos adicionais separadamente, para distribuição não-exclusiva da versão do trabalho publicada nesta revista (ex.: publicar em repositório institucional ou como capítulo de livro), com reconhecimento de autoria e publicação inicial nesta revista.
- Autores têm permissão e são estimulados a publicar e distribuir seu trabalho online (ex.: em repositórios institucionais ou na sua página pessoal) a qualquer ponto antes ou durante o processo editorial, já que isso pode gerar alterações produtivas, bem como aumentar o impacto e a citação do trabalho publicado (Veja O Efeito do Acesso Livre).