Classification of Accident Causes on Highways in Minas Gerais Using Machine Learning
Keywords:
Supervised Learning, K-Nearest Neighbor, Random Forest, Support Vector MachineAbstract
Road traffic accidents are one of the leading causes of global mortality, especially among young people and adults, and they have significant impacts on essential areas such as health and the economy. This study aims to compare and select a model to classify the causes of accidents on federal highways in Minas Gerais, Brazil, using data processing methods and Machine Learning techniques. Data from the Federal Highway Police, from January 1, 2023, to September 30, 2023, were used to analyze the Random Forest (RF), Support Vector Machine (SVM), and K-Nearest Neighbors (K-NN) algorithms. The SVM showed the best accuracy and Kappa index, while the RF had reasonable performance, and the K-NN was inferior and slower. The analysis reinforces the importance of carefully choosing the model, considering performance and computational efficiency. The study aims to support security authorities, such as the Federal Highway Police, in analyzing and recording occurrences, strengthening the construction of a robust and reliable database for filling out the Traffic Accident Report.
References
DETRAN-MG. Registro Nacional de Acidentes e Estatísticas de Trânsito. 2024. Disponível em: https://transito.mg.gov.br/sobre1/estatisticas/registro-nacional-de-acidentes-de-transito. Acesso em: 15 jan. 2024.
HOSSIN, M. N.; SULAIMAN A. Review on Evaluation Metrics for Data Classification Evaluations. International Journal Of Data Mining & Knowledge Management Process, [S.L.], v. 5, n. 2, p. 01-11, 31 mar. 2015. Academy and Industry Research Collaboration Center (AIRCC). http://dx.doi.org/10.5121/ijdkp.2015.5201
LIAW A, WIENER M (2022). Random Forest: Breiman and Cutler's Random Forests for Classification and Regression. R package version 4.7-1.1. https://cran.r-project.org/web/packages/randomForest/index.html
MALAQUIAS, E. O.; TOSTA, M. C. R.; CHAVES, G. L. D.; RIBEIRO, G. M. ACIDENTES EM RODOVIAS BRASILEIRAS: um estudo com técnicas de machine learning para classificar a causa das ocorrências. In: 35° CONGRESSO DE PESQUISA E ENSINO EM TRANSPORTE DA ANPET, 35., 2021, online. [S. I.]: Anpet, 2021. p. 2322-2334.
MEGNIDIO-TCHOUKOUEGNO, M; ADEDEJI, J. A. Machine Learning for Road Traffic Accident Improvement and Environmental Resource Management in the Transportation Sector. Sustainability, [S.L.], v. 15, n. 3, p. 2014, 20 jan. 2023. MDPI AG. http://dx.doi.org/10.3390/su15032014
MEYER, D.; DIMITRADOU, E.; HORNIK, K.; WEINGESSEL, A.; LEISCH, F. e1071: Misc Functions of the Department of Statistics, Probability Theory Group (Formerly: E1071), TU Wien. R package version 1.7-16, 2023. https://CRAN.R-project.org/package=e1071
MINAS GERAIS. SECRETARIA DE SAÚDE DE MINAS GERAIS. Acidentes por Transporte Terrestre. Disponível em: http://vigilancia.saude.mg.gov.br/index.php/paineis-tematicos/. Acesso em: 15 jan. 2024.
PRF (Polícia Rodoviária Federal). Dados Abertos da PRF. 2023. Disponível em: https://www.gov.br/prf/pt-br/acesso-a-informacao/dados-abertos/dados-abertos-da-prf. Acesso em: 12 jan. 2023.
R CORE TEAM. R: A language and environment for statistical computing. R Foundation for Statistical Computing, Vienna, Austria. 2023. ISBN 3-900051-07-0, URL http://www.R-project.org
RAINIO, O.; TEUHO, J.; KLÉN, R. Evaluation metrics and statistical tests for machine learning. Scientific Reports, [S.L.], v. 14, n. 1, p. 255-269, 13 mar. 2024. Springer Science and Business Media LLC. http://dx.doi.org/10.1038/s41598-024-56706-x
RIPLEY, B.; VENABLES, W. class: Functions for Classification. R package version 7.3-22, 2023. https://cran.r-project.org/web/packages/class/index.html
World Health Organization. Despite notable progress, road safety remains urgent global issue. 2023. https://www.who.int/news/item/13-12-2023-despite-notable-progress-road-safety-remains-urgent-global-issue. Acesso: 10 jan. 2024.
World Health Organization. Global status report on road safety 2023. 2023. Disponível em: https://www.who.int/teams/social-determinants-of-health/safety-and-mobility/global-status-report-on-road-safety-2023. Acesso em: 10 jan. 2024.
World Health Organization. Road safety Brazil 2023 country profile. 2023. Disponível em: https://www.who.int/publications/m/item/road-safety-bra-2023-country-profile. Acesso em: 10 jan. 2024.
XU, Haojie et al. PFD-Assisted Sampling PLL With Seamless PFD-SPD Switching Scheme and Supply-Insensitive RO. Ieee Microwave And Wireless Technology Letters, [S.L.], v. 33, n. 10, p. 1474-1477, out. 2023. Institute of Electrical and Electronics Engineers (IEEE). http://dx.doi.org/10.1109/lmwt.2023.3307733
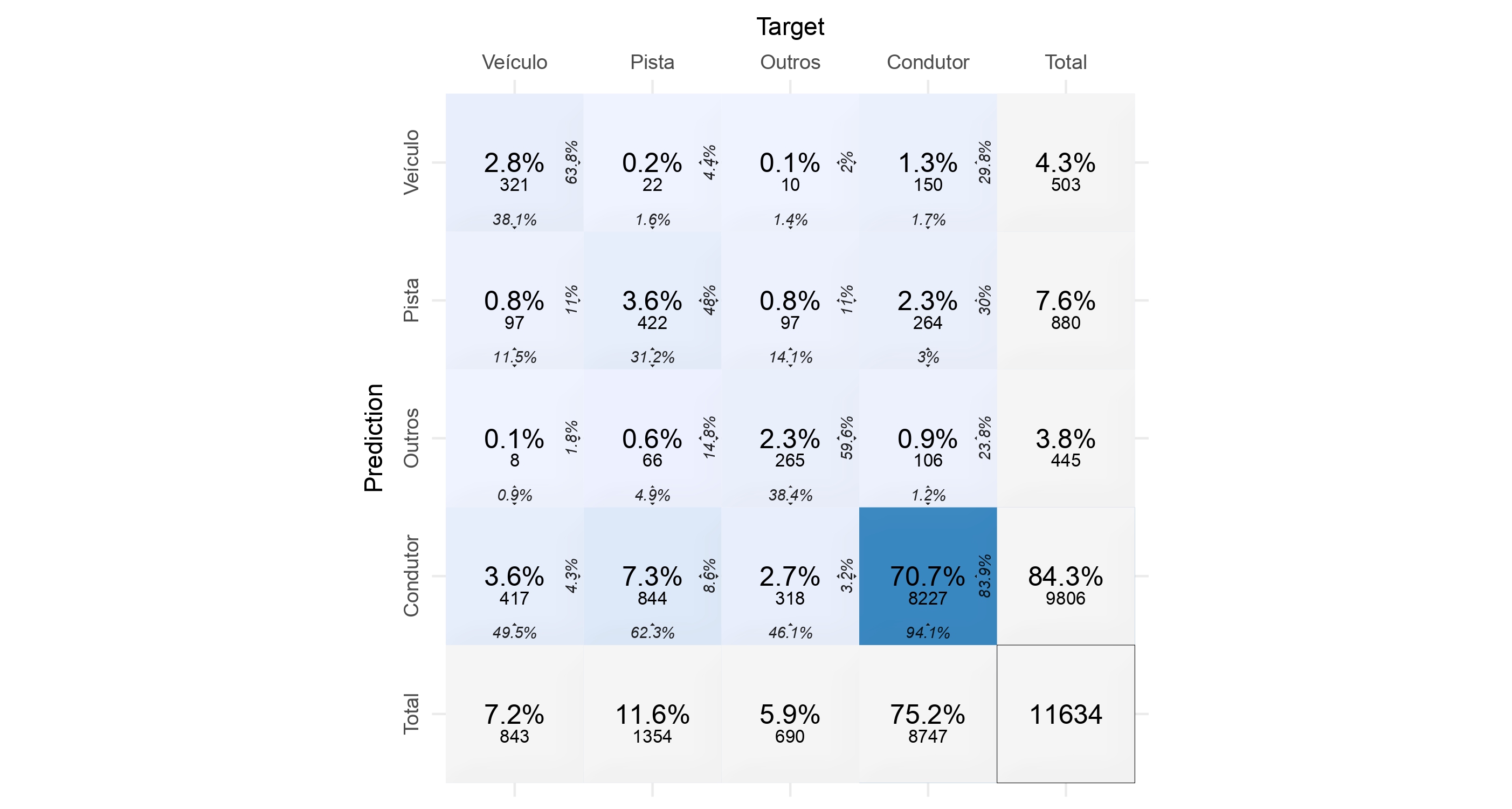
Downloads
Published
How to Cite
Issue
Section
License
Proposta de Política para Periódicos de Acesso Livre
Autores que publicam nesta revista concordam com os seguintes termos:
- Autores mantém os direitos autorais e concedem à revista o direito de primeira publicação, com o trabalho simultaneamente licenciado sob a Licença Creative Commons Attribution que permite o compartilhamento do trabalho com reconhecimento da autoria e publicação inicial nesta revista.
- Autores têm autorização para assumir contratos adicionais separadamente, para distribuição não-exclusiva da versão do trabalho publicada nesta revista (ex.: publicar em repositório institucional ou como capítulo de livro), com reconhecimento de autoria e publicação inicial nesta revista.
- Autores têm permissão e são estimulados a publicar e distribuir seu trabalho online (ex.: em repositórios institucionais ou na sua página pessoal) a qualquer ponto antes ou durante o processo editorial, já que isso pode gerar alterações produtivas, bem como aumentar o impacto e a citação do trabalho publicado (Veja O Efeito do Acesso Livre).