MACHINE LEARNING: PREDICTING CREDIT RISK
Keywords:
Machine Learning, Credit Risk, Financial Market, Default, Classification ModelsAbstract
The purpose of this article is to employ Machine Learning techniques to develop classification models applied to credit risk data. To achieve this goal, the R software was utilized, implementing various methodologies, including Logistic Regression, Bagging, Random Forest, and Decision Trees. Additionally, ensemble techniques were applied to combine these models, aiming to improve the accuracy of the predictions. The metric chosen for the comparative evaluation of the models was Accuracy and AUC (area under the curve). This study seeks to explore the effectiveness of these approaches in predicting and classifying credit risks, contributing to a deeper understanding and practical application of machine learning techniques in the financial context.
References
COELHO, F. F.; AMORIM, D. P. de L.; CAMARGOS, M. A. de. Analisando métodos de machine learning e avaliação do risco de crédito. Revista Gestão & Tecnologia, v. 21, n. 1, p. 89–116, 2021.
MOURAO, V. D. G.; CAJUEIRO, D. O. Estudo comparativo entre técnicas de machine learning para classificação do tomador PJ – MPE (Micro e Pequenas Empresas) [Dissertação de Mestrado, Universidade de Brasília].
IZBICKI, R.; SANTOS, T. M. dos. Aprendizado de máquina: uma abordagem estatística [livro eletrˆonico]. São Carlos, SP: Rafael Izbicki, 2020.
JAMES, G.; WITTEN, D.; HASTIE, T.; TIBSHIRANI, R. An Introduction to Statistical Learning with Applications in R (2nd ed.). Springer, 2023. ISBN 978-3-030-70396-1.
LOPES, L. P. Predicting the price of Brazilian Natural coffee using statistical machine learning models. Sigmae, v. 7, n. 1, p. 1–16, 2018. Disponível em: https://publicacoes.unifal-mg.edu.br/revistas/index.php/sigmae/article/view/699.
BENTO, C. E. A. Inadimplˆencia em tempos de pandemia: uma análise do crédito imobiliário. Dissertação de mestrado profissional, Fundação Getulio Vargas, Escola de Políticas Públicas e Governo, 2023.
BORDIN, I. T.; ROSSONI, D. F. Fatores associados `a percepção de prejuízo na aprendizagem de estudantes de universidades públicas brasileiras durante a pandemia de Covid-19. Sigmae, Programa de Pós-Graduação em Bioestatística, Universidade Estadual de Maringá (UEM), 2022.
SHI, S.; TSE, R.; LUO, W.; D’ADDONA, S.; PAU, G. Machine learning-driven credit risk: a systemic review, 2022.
BRODERSEN, K. H.; ONG, C. S.; STEPHAN, K. E.; BUHMANN, J. M. The balanced accuracy and its posterior distribution. In Proceedings of the 20th International Conference on Pattern Recognition, p. 3121-3124, IEEE, 2010.
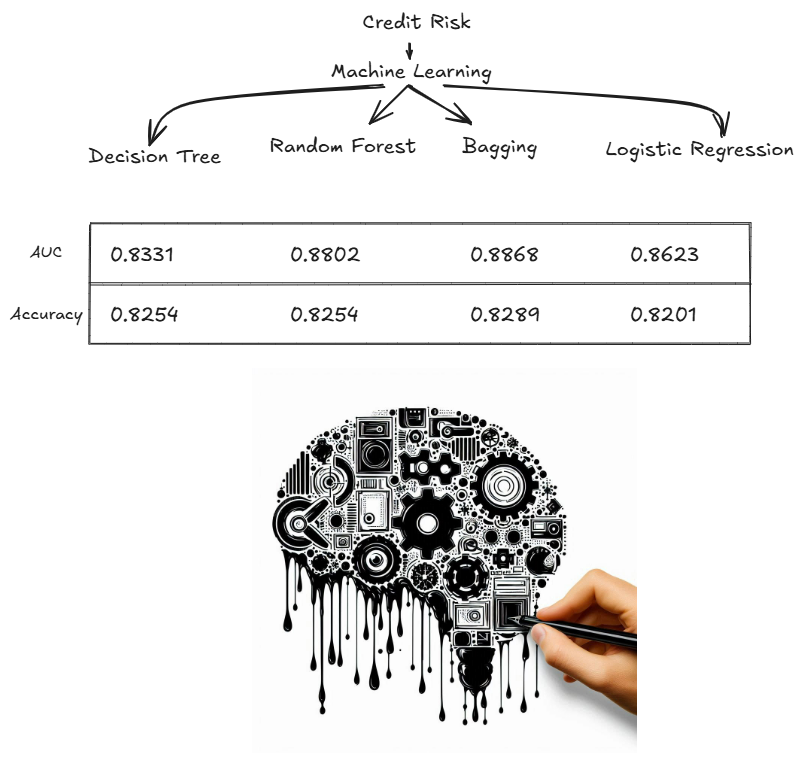
Downloads
Published
How to Cite
Issue
Section
License
Proposta de Política para Periódicos de Acesso Livre
Autores que publicam nesta revista concordam com os seguintes termos:
- Autores mantém os direitos autorais e concedem à revista o direito de primeira publicação, com o trabalho simultaneamente licenciado sob a Licença Creative Commons Attribution que permite o compartilhamento do trabalho com reconhecimento da autoria e publicação inicial nesta revista.
- Autores têm autorização para assumir contratos adicionais separadamente, para distribuição não-exclusiva da versão do trabalho publicada nesta revista (ex.: publicar em repositório institucional ou como capítulo de livro), com reconhecimento de autoria e publicação inicial nesta revista.
- Autores têm permissão e são estimulados a publicar e distribuir seu trabalho online (ex.: em repositórios institucionais ou na sua página pessoal) a qualquer ponto antes ou durante o processo editorial, já que isso pode gerar alterações produtivas, bem como aumentar o impacto e a citação do trabalho publicado (Veja O Efeito do Acesso Livre).