Regression tree for prediction the yield of fresh matter of teosinte aerial part in function of meteorological variables
Keywords:
Zea mays ssp. mexicana, global solar radiation, thermal sum, regression treeAbstract
The objective of this work was to verify if it is possible to predict the yield of fresh matter of teosinte aerial part in function of meteorological variables. An experiment was conducted with nine sowing dates (10/08/2021 to 01/01/2022). Sowings were carried out in a 5 m long row, spaced 0.80 m between rows and 0.20 m between plants in the row. In each row, five plants were randomly selected, totaling 45 plants. On 03/28/2022, in the first five sowing dates, and on 04/22/2022, in the last four sowing dates, the fresh matter of the aerial part of the plant (FMAP, in g) was determined. The cumulative global solar radiation and the thermal sum of the subperiods from sowing to male flowering (vegetative stage) and male flowering to harvest (reproductive stage) were calculated. The regression tree analysis algorithm was applied to forecast the FMAP as a function of meteorological variables. The regression tree was performed with data from all plants and sowing dates (n=45). The cumulative global solar radiation from male flowering to harvest was the main dividing point. In the second hierarchical node, the division criteria were thermal sum from sowing to male flowering and cumulative global solar radiation from sowing to male flowering. The highest productive performance (FMAP = 1162 g plant-1) was observed in plants with cumulative global solar radiation in the reproductive stage lower than 494 MJ m-2 and global accumulated solar radiation in the vegetative stage lower than 3257 MJ m-2 (33% of observations).
References
ALVARES, C. A. et al. Köppen’s climate classification map for Brazil. Meteorologische Zeitschrift, v. 22, n. 6, p. 711-728, 2013.
ARNOLD, C. Y. Maximum-minimum temperatures as a basis for computing heat units. Journal of the American Society for Horticultural Sciences, v. 76, n. 1, p. 682-692, 1960.
BRETAS, I. L. et al. Prediction of aboveground biomass and dry‐matter content in Brachiaria pastures by combining meteorological data and satellite imagery. Grass and Forage Science, v. 76, n. 3, p. 340-352, 2021.
COELHO, A. E. et al. Nitrogen use efficiency and grain yield of corn hybrids as affected by nitrogen rates and sowing dates in subtropical environment. Revista Brasileira de Ciência do Solo, v. 46, e0210087, p. 1-21, 2022.
DJAMAN, K. et al. Planting date and plant density effects on maize growth, yield and water use efficiency. Environmental Challenges, v. 6, e100417, p. 1-11, 2022.
JEONG, J. H. et al. Random forests for global and regional crop yield predictions. Plos One, v. 11, n. 6, e0156571, 2016.
JHA, A. K. et al. Impact of irrigation method on water use efficiency and productivity of fodder crops in Nepal. Climate, v. 4, n. 1, p. 1-13, 2016.
KUMAR, B. et al. Herbage production, nutritional composition and quality of teosinte under Fe fertilization. International Journal of Agriculture & Biology, v. 18, n. 2, p. 319-329, 2016.
LOH, W. Y. Classification and regression trees. Wiley Interdisciplinary Reviews: Data Mining and Knowledge Discovery, v. 1, n. 1, p. 14-23, 2011.
MILBORROW, S. _rpart.plot: Plot ´rpart` Models: an Enhanced Version of ´plot.rpart`_. R package version 3.1.1, 2022. <https://CRAN.R-project.org/package=rpart.plot>.
MOHAN, S.; DAR, E. A.; SINGH, M. Fodder quality of teosinte fodder as influenced by nitrogen, phosphorus and zinc application. International Journal of Pure and Applied Bioscience, v. 5, p. 596-604, 2017.
R CORE TEAM. R: A Language and Environment for Statistical Computing. R Foundation for Statistical Computing, Vienna, Austria, 2023. Disponível em: https://www.R-project.org/.
SANTOS, H. G. et al. Sistema Brasileiro de Classificação de Solos. 5. ed. Brasília: Embrapa. p. 356, 2018.
THERNEAU, T.; ATKINSON, B. _rpart: Recursive Partitioning and Regression Trees_. R package version 4.1.19, 2022. Disponível em: https://CRAN.R-project.org/package=rpart.
YANG, Y. S. et al. The effect of solar radiation change on the maize yield gap from the perspectives of dry matter accumulation and distribution. Journal of Integrative Agriculture, v. 20, n. 2, p. 482-493, 2021.
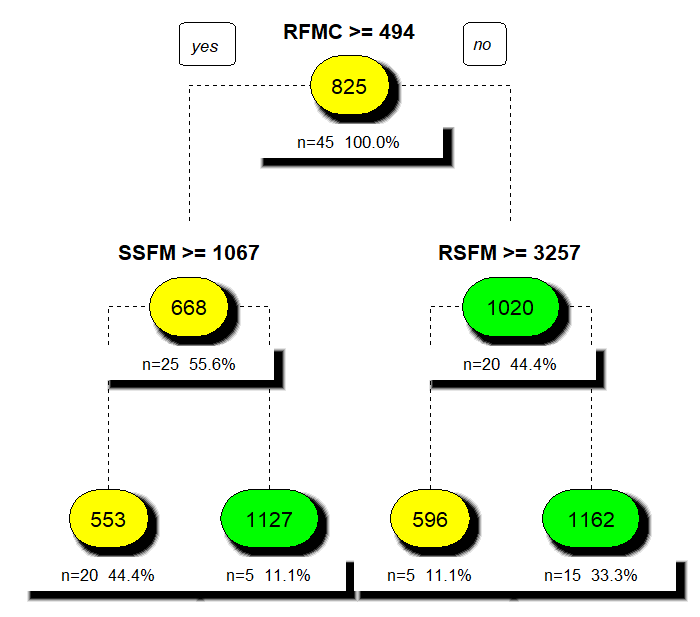
Downloads
Published
How to Cite
Issue
Section
License
Proposta de Política para Periódicos de Acesso Livre
Autores que publicam nesta revista concordam com os seguintes termos:
- Autores mantém os direitos autorais e concedem à revista o direito de primeira publicação, com o trabalho simultaneamente licenciado sob a Licença Creative Commons Attribution que permite o compartilhamento do trabalho com reconhecimento da autoria e publicação inicial nesta revista.
- Autores têm autorização para assumir contratos adicionais separadamente, para distribuição não-exclusiva da versão do trabalho publicada nesta revista (ex.: publicar em repositório institucional ou como capítulo de livro), com reconhecimento de autoria e publicação inicial nesta revista.
- Autores têm permissão e são estimulados a publicar e distribuir seu trabalho online (ex.: em repositórios institucionais ou na sua página pessoal) a qualquer ponto antes ou durante o processo editorial, já que isso pode gerar alterações produtivas, bem como aumentar o impacto e a citação do trabalho publicado (Veja O Efeito do Acesso Livre).