Factors associated with depression in pregnant women during the COVID-19 pandemic
Keywords:
Beta distribution, Distributional regression, GAMLSS, Zero inflationAbstract
Pregnancy is a challenging period for women, especially during the pandemic, which saw a large increase in occurrences of depression among pregnant mothers. In light of this, the purpose of this study is to discover the features that contributed to depression in pregnant women at this time. The following risk factors were taken into account: the mother’s age, annual family income, maternal education, anxiety level, gestational age, concern about virus exposure, concerns about hurting the baby’s health, and the baby’s life. In this dataset of 6,162 observations, the response variable depression was measured using the Edinburgh Postnatal Depression Scale (EDPS), a self assessment questionnaire developed in the United Kingdom for postpartum depression research, and transformed into a scale of zero to one, where a value of zero indicates the absence of any signs or manifestations associated with depression and a value of one indicates the maximum presence of depressive signs. Thus, generalised additive models for location, scale, and shape (GAMLSS) based on the zero-inflated beta distribution were considered. Covariates were selected for each of the distribution parameters using a stepwise-based method, allowing us to detect the primary features that lead to a woman scoring zero on the EDPS, as well as the main risk factors for developing depression. The residual analysis indicated that the fitted model was adequate for describing the analysed data. This study contributes to a better knowledge of the factors that influence pregnant women’s mental health throughout the pandemic, generating recommendations for public health strategies.
References
AKAIKE, H. A new look at the statistical model identification. IEEE Transactions on Automatic Control, v. 19, p. 716--723, 1974.
AYAZ, R.; HOCAOGLU, M.; GUNAY, T.; YARDIMCI, O.; TURGUT, A.; KARATEKE, A. Anxiety and depression symptoms in the same pregnant women before and during the COVID-19 pandemic. Journal of Perinatal Medicine, v. 48, p. 965--970, 2020.
COX, J. L.; HOLDEN, J. M.; SAGOVSKY, R. Detection of postnatal depression. Development of the 10-item Edinburgh Postnatal Depression Scale. The British Journal of Psychiatry, v. 150, p. 782--786, 1987.
DE BASTIANI, F.; RIGBY, R. A.; STASINOPOULOS, D. M.; CYSNEIROS, A. H. M. A.; URIBE-OPAZO, M. A. Gaussian Markov random field spatial models in GAMLSS. Journal of Applied Statistics, v. 45, p. 168--186, 2018.
DUNN, P. K.; SMYTH, G. K. Randomized quantile residuals. Journal of Computational and Graphical Statistics, v. 5, p. 236--244, 1996.
EILERS, P. H. C.; MARX, B. D. Flexible smoothing with B-splines and penalties. Statistical Science, v. 11, p. 89--121, 1996.
HASTIE, T. J.; TIBSHIRANI, R. J. Generalized Additive Models. CRC Press, 1990.
KING, L. S.; FEDDOES, D. E.; KIRSHENBAUM, J. S.; HUMPHREYS, K. L.; GOTLIB, I. H. Pregnancy during the pandemic: the impact of COVID-19-related stress on risk for prenatal depression. Psychological Medicine, v. 53, n. 1, p. 170--180, 2023.
LEBEL, C.; TOMFOHR-MADSEN, L.; GIESBRECHT, G.; LAI, B.P.Y.; BAGSHAWE, M.; FREEMAN, M.; HAPIN, M.K.; MACKINNON, A.; PATEL, P.; VAN SLOTEN, M.; VAN DE WOUW, M. Prenatal mental health data and birth outcomes in the pregnancy during the COVID-19 Pandemic dataset. Data in Brief, v. 49, p. 109366, 2023.
LEE, J. D.; SUN, D. L.; SUN, Y.; TAYLOR, J. E. Exact post-selection inference, with application to the lasso. The Annals of Statistics, v. 44, p. 907--927, 2016.
NAKAMURA, L. R.; RIGBY, R. A.; STASINOPOULOS, D. M.; LEANDRO, R. A.; VILLEGAS, C.; PESCIM, R. R. Modelling location, scale and shape parameters of the Birnbaum-Saunders generalized $t$ distribution. Journal of Data Science, v. 15, p. 221--237, 2017.
NELDER, J. A.; WEDDERBURN, R. W. M. Generalized Linear Models. Journal of the Royal Statistical Society. Series A: Statistics in Society, v. 135, p. 370--384, 1972.
PAIXÃO, G. P. N.; CAMPOS, L. M.; CARNEIRO, J. B.; FRAGA, C. D. S. A solidão materna diante das novas orientações em tempos de SARS-COV-2: um recorte brasileiro. Revista Gaúcha de Enfermagem, v. 42, p. e20200165, 2021.
RABINOWITZ, E. P.; KUTASH, L. A.; RICHESON, A. L.; SAYER, M. A.; SAMII, M. R.; DELAHANTY, D. L. Depression, anxiety, and stress in pregnancy and postpartum: A longitudinal study during the COVID-19 pandemic. Midwifery, v. 121, p. 103655, 2023.
RAMIRES, T. G.; NAKAMURA, L. R.; RIGHETTO, A. J.; PESCIM, R. R.; MAZUCHELI, J.; CORDEIRO, G. M. A new semiparametric Weibull cure rate model: fitting different behaviors within GAMLSS. Journal of Applied Statistics, v. 46, p. 2744--2760, 2019.
RAMIRES, T. G.; NAKAMURA, L. R.; RIGHETTO, A. J.; PESCIM, R. R.; MAZUCHELI, J.; RIGBY, R. A.; STASINOPOULOS, D. M. Validation of Stepwise-Based Procedure in GAMLSS. Journal of Data Science, v. 19, p. 96--110, 2021.
RIGBY, R.A.; STASINOPOULOS, M.D.; HELLER, G.Z.; DE BASTIANI, F. Distributions for Modeling Location, Scale, and Shape: Using GAMLSS in R}. Boca Raton: CRC Press, 2019.
RIGBY, R.A.; STASINOPOULOS, M.D., Generalized Additive Models for Location, Scale and Shape. Journal of the Royal Statistical Society. Series C: Applied Statistics, v. 54, p. 507--554, 2005.
STASINOPOULOS, M.D.; RIGBY, R.A.; DE BASTIANI, F. GAMLSS: A distributional regression approach. Statistical Modelling, v. 18, p. 248--273, 2018.
STASINOPOULOS, M.D.; RIGBY, R.A.; HELLER, G.Z.; VOUDOURIS, V.; DE BASTIANI, F. Flexible Regression and Smoothing: Using GAMLSS in R. Chapman and Hall/CRC, 2017.
VAN BUUREN, S.; FREDRIKS, M. Worm plot: a simple diagnostic device for modelling growth reference curves. Statistics in Medicine, v. 20, n. 8, p. 1259--1277, 2001.
WHO (World Health Organization). Depression and Other Common Mental Disorders: Global Health Estimates. 2017.
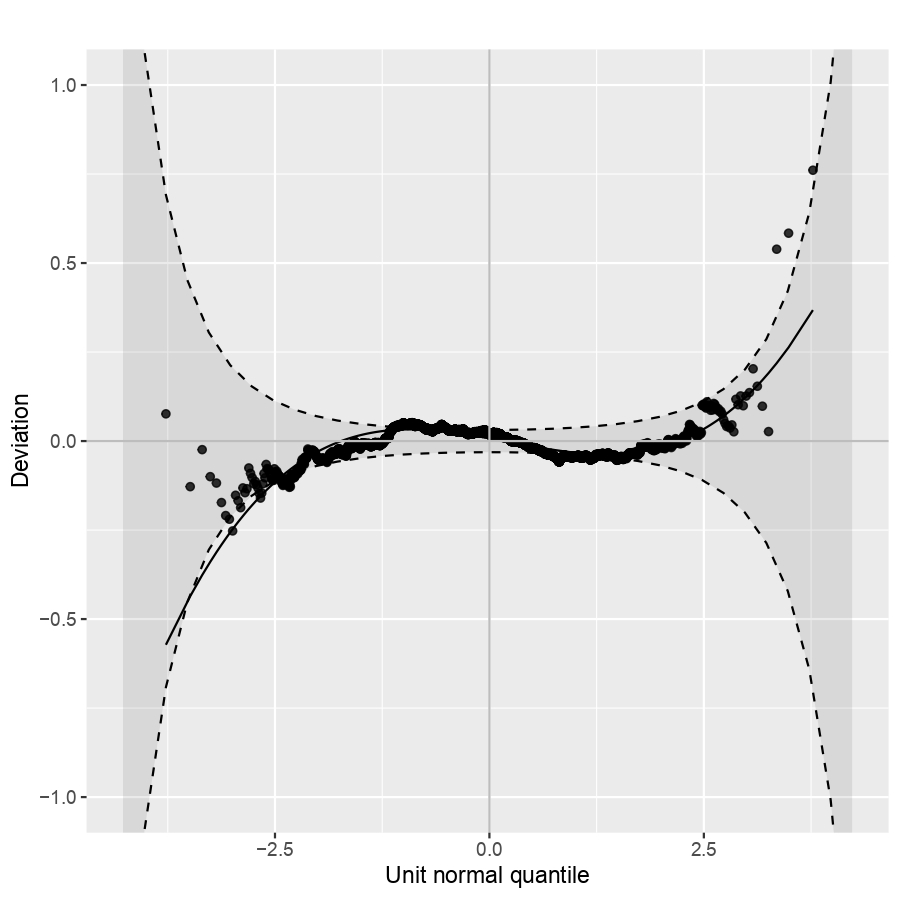
Downloads
Published
How to Cite
Issue
Section
License
Proposta de Política para Periódicos de Acesso Livre
Autores que publicam nesta revista concordam com os seguintes termos:
- Autores mantém os direitos autorais e concedem à revista o direito de primeira publicação, com o trabalho simultaneamente licenciado sob a Licença Creative Commons Attribution que permite o compartilhamento do trabalho com reconhecimento da autoria e publicação inicial nesta revista.
- Autores têm autorização para assumir contratos adicionais separadamente, para distribuição não-exclusiva da versão do trabalho publicada nesta revista (ex.: publicar em repositório institucional ou como capítulo de livro), com reconhecimento de autoria e publicação inicial nesta revista.
- Autores têm permissão e são estimulados a publicar e distribuir seu trabalho online (ex.: em repositórios institucionais ou na sua página pessoal) a qualquer ponto antes ou durante o processo editorial, já que isso pode gerar alterações produtivas, bem como aumentar o impacto e a citação do trabalho publicado (Veja O Efeito do Acesso Livre).