COVID-19 pandemic in the municipalities of the State of Paraná
an investigation via Cluster Analysis
Keywords:
Cluster analysis, Clusters, J48, Naive Bayes, Covid-19Abstract
This research addresses the epidemiological context of the COVID-19 pandemic, emphasizing the specific panorama of Brazil, with a special focus on the State of Paran´a, approximately one year after the implementation of the first lockdown. The general objective of this research is to apply the clustering technique known as k-means to categorize municipalities in Paran´a based on two main variables: the daily number of confirmed cases and the number of deaths from COVID-19. To achieve this purpose, data provided by the Parana´ Department of Health were used, covering the period from January 1, 2021 to March 15, 2021. The results obtained revealed the identification of three clusters that stood out as excellent , highlighting divergences in the incidence patterns of cases and deaths between municipalities. Notably, a correlation was observed between population density and the frequency of cases and deaths, with more densely populated areas tending to record higher numbers. Furthermore, the evaluation of the accuracy of the J48 and Naive Bayes algorithms in classifying clusters demonstrated satisfactory results. Consequently, it is concluded that the grouping technique used proved to be effective in identifying similarities in the spread patterns of COVID-19, offering relevant evidence for the formulation of targeted and efficient strategies to combat the pandemic, especially in the most impacted regions.
References
ALVES, H. J. P.; FERNANDES, F. A.; LIMA, K. P.; BATISTA, B. D. O.; FERNANDES, T. J. A pandemia da COVID-19 no Brasil: uma aplicação do método de clusterização k-means. Research, Society and Development, v. 9, n. 10, 2020. DOI: http://dx.doi.org/10.33448/rsd-v9i10.9059.
CHARRAD, M., GHAZZALI, N., BOITEAU, V., NIKNAFS, A. Determining the best number of clusters in a data set. Package NbClust, 2015. Recuperado de http://cran.rediris.es/ web/packages/NbClust/NbClust.pdf.
EMAMI, A.; JAVANMARDI, F.; PIRBONYEH, N.; AKBARI, A. Prevalence of Underlying Diseases in Hospitalized Patients with COVID-19: a Systematic Review and Meta-Analysis. Arch Acad Emerg Med. 8(1): e35, mar, 2020.
FAVERO, L. P.; BELFIORE, P. Data Science for Business and Decision Making. Academic Press, Cambridge, MA, USA, 2019
FAYYAD, U.M. et al. Advances in knowledge discovery and data mining. Massachusetts: AAAI Press, 1996.
GUIMARAES, R. M.; ELEUTERIO, T. D. A.; MONTEIRO-DA-SILVA, J. H. C. Estratificação de risco para predição de disseminação e gravidade da Covid-19 no Brasil. Revista Brasileira De Estudos De População, 37, 1-17, 2020. DOI:
http://dx.doi.org/10.20947/s0102- 3098a0122.
IRITANI, O.; OKUNO, T.; HAMA, D.; KANE, A.; KODERA, K.; MORIGAKI, K.; TERAI, T.; MAENO, N.; MORIMOTO, S. Clusters of covid-19 in long-term care hospitals and facilities in japan from 16 january to 9 may 2020. Geriatrics & gerontology international, 20(7), 715-719, 2020. DOI: 10.1111/ggi.13973.
JAMES, N.; MENZIES, M. Cluster-based dual evolution for multivariate time series: Analyzing covid-19. Chaos: An Interdisciplinary Journal of Nonlinear Science, 30, 2020. DOI: https://doi.org/10.1063/5.0013156.
LU, R.; ZHAO, X.; LI, J.; NIU, P.; YANG, B.; WU, H.; WANG, W. Genomic characterisation and epidemiology of 2019 novel coronavirus: implications for virus origins and receptor binding. The Lancet. v.395, Feb 22, P. 565-574, 2020.
https://doi.org/10.1016/S0140-6736(19)33096-X.
MACIEL, E. L.; JABOR, P.; GONC¸ ALVES JUNIOR, E.; TRISTÃO-SÁ, R.; LIMA, R.C.D.; REIS-SANTOS, B.; LIRA, P.; BUSSINGUER, E. C. A.; ZANDONADE, E. Fatores associados ao óbito hospitalar por covid-19 no Espírito Santo. Epidemiologia e Serviços de Saúde, 29(4), 1-11, 2020. DOI: 10.5123/S1679-49742020000400022.
R CORE TEAM. R: A language and environment for statistical computing. R Foundation for Statistical computing, Vienna, 2020. Dispon´ıvel em: https://www.Rproject.org/.
RATKOWSKY, D.; LANCE, G. Criterion for determining the number of groups in a classification. Australian Computer Journal, 10(3), 115-117, 1978.
SESA, Secretaria da saúde: Informe Epidemiológico Coronavírus (COVID-19). Boletim epidemiológico, Curitiba, Março, 2021. Dispon ́ıvel em:
https://www.saude.pr.gov.br/sites/default/arquivos restritos/files/documento/2021-03/informe epidemiológico 15 03 2021.pdf.
WITTEN IH, F. Data Mining: Practical Machine Learning Tools and Techniques. 2nd edition. Morgan Kaufmann, San Francisco, 2005.
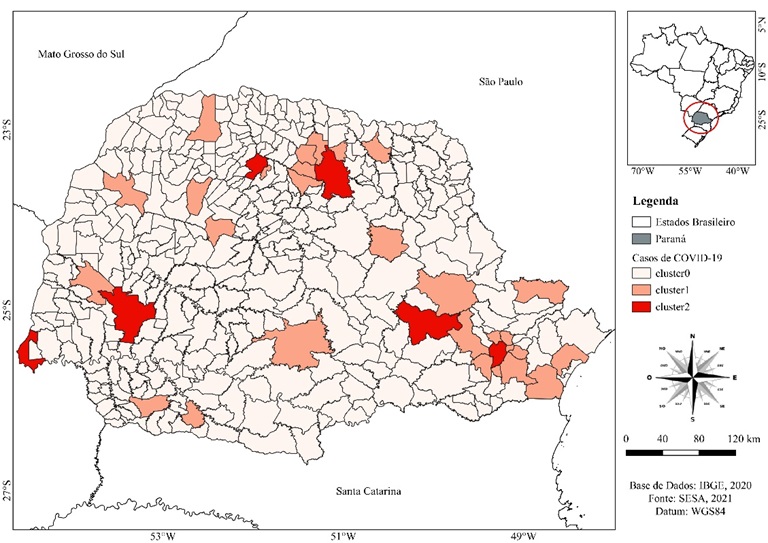
Downloads
Published
How to Cite
Issue
Section
License
Proposta de Política para Periódicos de Acesso Livre
Autores que publicam nesta revista concordam com os seguintes termos:
- Autores mantém os direitos autorais e concedem à revista o direito de primeira publicação, com o trabalho simultaneamente licenciado sob a Licença Creative Commons Attribution que permite o compartilhamento do trabalho com reconhecimento da autoria e publicação inicial nesta revista.
- Autores têm autorização para assumir contratos adicionais separadamente, para distribuição não-exclusiva da versão do trabalho publicada nesta revista (ex.: publicar em repositório institucional ou como capítulo de livro), com reconhecimento de autoria e publicação inicial nesta revista.
- Autores têm permissão e são estimulados a publicar e distribuir seu trabalho online (ex.: em repositórios institucionais ou na sua página pessoal) a qualquer ponto antes ou durante o processo editorial, já que isso pode gerar alterações produtivas, bem como aumentar o impacto e a citação do trabalho publicado (Veja O Efeito do Acesso Livre).