Shrinkage effect in the Bayesian analysis of the GGE model using maximum entropy prior
Keywords:
GGE model, Bayesian inference, Maximum entropyAbstract
In this work, the maximum entropy principle was used to assign a priori information to the variance components of the singular values in the genotype main effects model plus genotype×environment interaction (GGE). The method was exemplified from simulated data. The results showed that the GGE model with maximum entropy priori (BGGEE) produces a shrinkage effect on the estimates of singular values, when compared with the frequentist GGE analysis or with the Bayesian GGE version that uses non-informative priors (referred to by BGGE). The BGGEE showed greater parsimony, estimating the singular values with greater contribution to the interaction effect and shrinking the estimates of singular values associated with larger dimensions to zero. Thus, BGGEE captured more pattern and discarded more noise than the typical Bayesian version. When using maximum entropy priori, it was found that the complete model and the one with only two bilinear terms are almost indistinguishable. This signals that model selection in the BGGEE fit would not be a necessary step. The method also avoids sampling problems observed when Jeffreys prior are used, resulting in proper and unimodal posterior marginal distributions.
References
BURGUEÑO, J. et al. Using factor analytic models for joining environments and genotypes without crossover genotype _ environment interaction. Crop Science, v. 48, n. 4, p. 1291-1305, 2008.
CHEN, M. H.; SHAO, Q. M. Monte Carlo estimation of Bayesian credible and HPD intervals. Journal of Computational and Graphical Statistics, v. 8, n. 1, p. 69-92, 1999.
CORNELIUS, P.; SEYEDSADR, M. Estimation of general linear-bilinear models for two-way tables. Journal of Statistical Computation and Simulation, v. 58, n. 4, p. 287-322, 1997.
CORNELIUS, P.; CROSSA, J.; SEYEDSADR, M. Statistical tests and estimators of multiplicative models for genotype-by-environment interaction. In:-------- . Genotype-byenvironment interaction. Boca Raton: CRC, 1996. p. 199-234.
CORNELIUS, P. L.; CROSSA, J. Prediction assessment of shrinkage estimators of multiplicative models for multi-environment cultivar trials. Crop Science, v. 39, n. 4, p. 998-1009, 1999.
CORNELIUS, P. Statistical tests and retention of terms in the additive main effects and multiplicative interaction model for cultivar trials. Crop Science, v. 33, n. 6, p. 1186-1193, 1993.
CROSSA, J.; YANG, R.-C.; CORNELIUS, P. L. Studying crossover genotype environment interaction using linear-bilinear models and mixed models. Journal of Agricultural, Biological, and Environmental Statistics, v. 9, n. 3, p. 362-380, 2004.
CROSSA, J. et al. Bayesian estimation of the additive main effects and multiplicative interaction model. Crop Science, v. 51, n. 4, p. 1458-1469, 2011.
CRUZ, C.; REGAZZI, A.; CARNEIRO, P. C. Modelos biométricos aplicados ao melhoramento genético. 4. ed. Viçosa: UFV, 2012. 514 p.
CROSSA, J.; CORNELIUS, P. L. Sites regression and shifted multiplicative model clustering of cultivar trial sites under heterogeneity of error variances. Crop Science, v. 37, n. 2, p. 406-415, 1997.
DENIS, J.-B.; GOWER, J. C. Asymptotic covariances for the parameters of biadditive models. Utilitas Mathematica, v. 46, p. 193-205, 1994.
HEIDELBERGER, P.; WELCH, P. D. Simulation run length control in the presence of an initial transient. Operations Research, v. 31, n. 6, p. 1109-1144, 1983.
HU, Z.; YANG, R. Improved statistical inference for graphical description and
interpretation of genotype times environment interaction. Crop Science, v. 53, n. 6, p.2400-2410, 2013.
JARQUÍN, D. et al. A hierarchical Bayesian estimation model for multi-environment plant breeding trials in successive years. Crop Science, v. 56, n.5, p. 2260-2276, 2016.
JAYNES, E. T. Information theory and statistical mechanics. Physical Review, APS, v. 106, n. 4, p. 620-630, 1957.
JAYNES, E. T. Probability theory: The logic of science. New York: Cambridge University Press, 2003. 757 p.
JEFFREYS, H. Theory of Probability. 3. ed. Oxford: Oxford Classic Texts in the Physical Sciences, 1961. 459 p.
LIU, G. Bayesian computations for general linear-bilinear models. 2001. 169 p. Thesis (Doctor of Philosophy) - University of Kentucky, Lexington, 2001.
OLIVEIRA, L. A. et al. Credible intervals for scores in the AMMI with random effects for genotype. Crop Science, v. 55, n. 2, p. 465-476, 2015.
OLIVEIRA, L. A. de et al. Bayesian GGE biplot models applied to maize multienvironments trials. Genetics and Molecular Research, v. 15, n. 2, p. 1-21, 2016.
PEREZ-ELIZALDE, S.; JARQUIN, D.; CROSSA, J. A general Bayesian estimation method of linear–bilinear models applied to plant breeding trials with genotype×environment interaction. Journal of Agricultural, Biological, and Environmental Statistics, v. 17, n. 1, p. 15-37, 2012.
R CORE TEAM. R: A language and environment for statistical computing. R Foundation for Statistical Computing, Vienna, Austria. 2019. URL: http://www.R-project.org/
RAFTERY, A. E. et al. Estimating the integrated likelihood via posterior simulation using the harmonic mean identity. In: ____. Bayesian Statistics. [S.l.], 2007. v. 8, p. 1-45.
RAFTERY, A. E.; LEWIS, S. M. [Practical Markov Chain Monte Carlo]: comment: one long run with diagnostics: implementation strategies for Markov Chain Monte Carlo. Statistical Science, v. 7, n. 4, p. 493-497, 1992.
ROMÃO, R. F. et al. Predictive ability of AMMI and factorial analytical models in the study of unbalanced multi-environment data. Genetics and Molecular Research, v. 18, n. 3, p. 1-20, 2019.
SILVA, C. P. et al. A Bayesian Shrinkage approach for AMMI Models. PLoS One, v.10, n. 7, p. e0131414, 2015.
SILVA, C. P. et al. Heterogeneity of variances in the Bayesian AMMI model for multienvironment trial studies. Crop Science, v. 59, n. 6, p. 2455-2472, 2019.
TER BRAAK, C.J.F; BOER, M.P.; BINK, M. C.A.M. Extending Xu's Bayesian model for estimating polygenic effects using markers of the entire genome. Genetics, v. 170, n. 3, p. 1435-1438, 2005.
VIELE, K.; SRINIVASAN, C. Parsimonious estimation of multiplicative interaction in analysis of variance using Kullback-Leibler information. Journal of Statistical Planning and Inference, v. 84, n. 1-2, p. 201-219, 2000.
YAN, W.; GLOVER, K. D.; KANG, M. S. Comment on “biplot analysis of genotype _environment interaction: proceed with caution” by R.-C. Yang, J. Crossa, P.L. Cornelius, and J. Burgueño in 2009, v.49, n.5: 1564-1576. Crop Science, v. 50, n. 4, p. 1121-1123, 2010.
YANG, R.-C. et al. Biplot analysis of genotype _ environment interaction: proceed with caution. Crop Science, v. 49, n. 5, p. 1564-1576, 2009.
YAN, W. Crop Variety Trials: Data Management and Analysis. New York: John Wiley & Sons, 2014. 361 p.
YAN, W et al. Cultivar evaluation and mega-environment investigation based on the GGE biplot. Crop Science, v. 40, n. 3, p. 597-605, 2000.
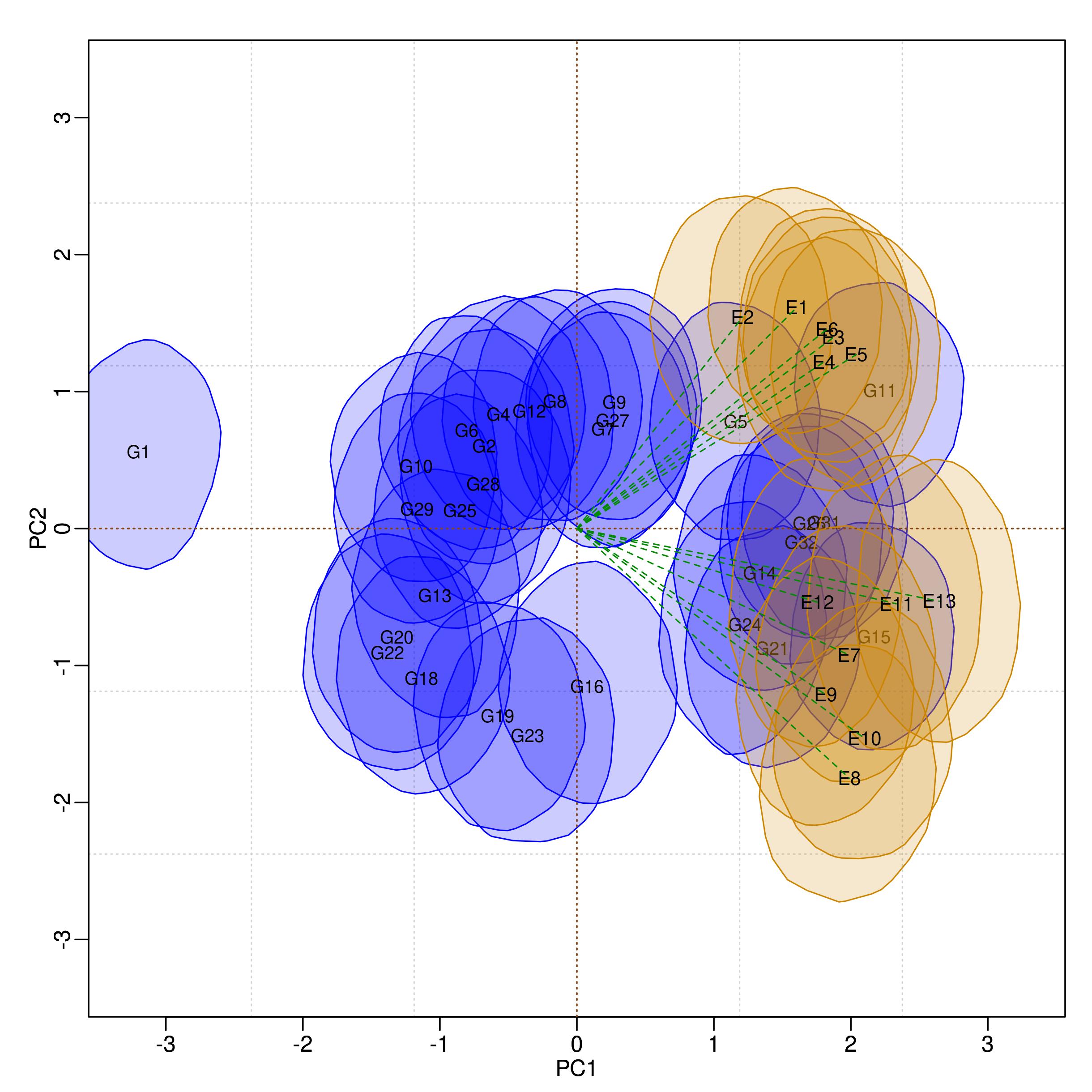
Downloads
Published
How to Cite
Issue
Section
License
Proposta de Política para Periódicos de Acesso Livre
Autores que publicam nesta revista concordam com os seguintes termos:
- Autores mantém os direitos autorais e concedem à revista o direito de primeira publicação, com o trabalho simultaneamente licenciado sob a Licença Creative Commons Attribution que permite o compartilhamento do trabalho com reconhecimento da autoria e publicação inicial nesta revista.
- Autores têm autorização para assumir contratos adicionais separadamente, para distribuição não-exclusiva da versão do trabalho publicada nesta revista (ex.: publicar em repositório institucional ou como capítulo de livro), com reconhecimento de autoria e publicação inicial nesta revista.
- Autores têm permissão e são estimulados a publicar e distribuir seu trabalho online (ex.: em repositórios institucionais ou na sua página pessoal) a qualquer ponto antes ou durante o processo editorial, já que isso pode gerar alterações produtivas, bem como aumentar o impacto e a citação do trabalho publicado (Veja O Efeito do Acesso Livre).