Monte Carlo evaluation of the ANOVA's F and Kruskal-Wallis tests under binomial distribution
Keywords:
Power, Type I error rate, Monte Carlo Simulation, CRDAbstract
To verify the equality of more than two levels of a factor under interest in experiments conducted under a completely randomized design (CRD) it is common to use the F ANOVA test, which is considered the most powerful test for this purpose. However, the reliability of such results depends on the following assumptions: additivity of effects, independence, homoscedasticity and normality of the errors. The nonparametric Kruskal-Wallis test requires more moderate assumptions and therefore it is an alternative when the assumptions required by the F test are not met. However, the stronger the assumptions of a test, the better its performance. When the fundamental assumptions are met the F test is the best option. In this work, the normality of the errors is violated. Binomial response variables are simulated in order to compare the performances of the F and Kruskal-Wallis tests when one of the analysis of variance assumptions is not satisfied. Through Monte Carlo simulation, were simulated $3,150,000$ experiments to evaluate the type I error rate and power rate of the tests. In most situations, the power of the F test was superior to the Kruskal-Wallis and yet, the F test controlled the Type I error rates.
References
CAMPOS, H. de. Estatística experimental não-paramétrica. 4a ed. Piracicaba: FEALQ, 1983.349p.
FEIR, B.; TOOTHAKER, L. The ANOVA F-test versus the Kruskal-Wallis test: a robustness study. In: Annual Meeting of the American Educational Research Association, Chicago, 1974. URL http://eric.ed.gov/ERICWebPortal/search/detailmini.jsp?_nfpb=true&_&ERICExtSearch_SearchValue_0=ED091423&ERICExtSearch_Search$ Chicago, 1974.
KRUSKAL, W. H; WALLIS, W. A. Use of ranks in one-criterion variance analysis, Journal of the American Statistical Association, Washington, v. 47, p. 583-621, 1952.
LIMA, P. C.; ABREU, A. R. de. Estatística experimental: ensaios balanceados. Lavras:
UFLA, 2000. 99 p.
R CORE TEAM. R: A language and environment for statistical computing. R Foundation for Statistical Computing, Vienna, Austria, 2011. ISBN 3-900051-07-0, URL http://www.R-project.org/.
REIS, G. M.; RIBEIRO, J. I. Jr. Comparação de testes paramétricos e não paramétricos aplicados em delineamentos experimentais. In: III Simpósio Acadêmico de Engenharia de Produção, Vicosa, 2007.Anais do III Simpósio Acadêmico de Engenharia de Produção, URL http:www.http://www.saepro.ufv.br/Image/artigos/SA03.pdf, Vicosa, 2007.
SIEGEL, S.; CASTELLAN, N. J. Jr. Estatística não-paramétrica para ciências do comportamento. 2 ed. Trad. S. I. C. Carmona. Porto Alegre: Artmed, 2006. 448p.
VIEIRA, S. Analise de variância (ANOVA). São Paulo: Atlas, 2006. 204p.
Sigmae, Alfenas,
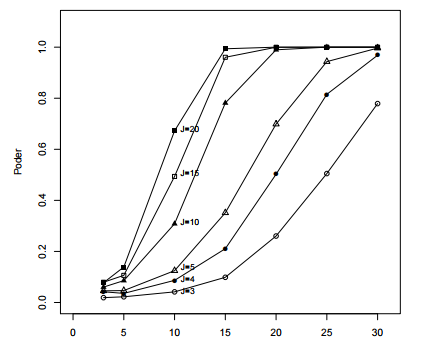
Downloads
Published
How to Cite
Issue
Section
License
Proposta de Política para Periódicos de Acesso Livre
Autores que publicam nesta revista concordam com os seguintes termos:
- Autores mantém os direitos autorais e concedem à revista o direito de primeira publicação, com o trabalho simultaneamente licenciado sob a Licença Creative Commons Attribution que permite o compartilhamento do trabalho com reconhecimento da autoria e publicação inicial nesta revista.
- Autores têm autorização para assumir contratos adicionais separadamente, para distribuição não-exclusiva da versão do trabalho publicada nesta revista (ex.: publicar em repositório institucional ou como capítulo de livro), com reconhecimento de autoria e publicação inicial nesta revista.
- Autores têm permissão e são estimulados a publicar e distribuir seu trabalho online (ex.: em repositórios institucionais ou na sua página pessoal) a qualquer ponto antes ou durante o processo editorial, já que isso pode gerar alterações produtivas, bem como aumentar o impacto e a citação do trabalho publicado (Veja O Efeito do Acesso Livre).