A comparison of multiple imputation methods for the analysis of survival data with outcome related missing covariate values
Keywords:
Missing covariates, Cox regression, multiple imputation, simulation study, censoring-ignorable MARAbstract
The Cox proportional hazards model is commonly used in medical research for investigating the association between the survival time and covariates. However, it is quite common for the analysis to involve missing covariate values. A reasonable assumption is that data are censoring-ignorable MAR in the sense that missingness does not depend on censoring time but may depend on failure time. In this case, a complete cases analysis produce biased regression coefficient estimates. Through a simulation study, we compare three multiple imputation approaches for a missing covariate when missingness is survival time-dependent: (i) the method proposed by White & Royston (2009) that uses the cumulative hazard in an approximation to the imputation model, (ii) the method described by Bartlett et al. (2015) that incorporates the Cox model in the imputation process, and (iii) the CART approach, a method known to deal with skewed distributions, interaction and nonlinear relations. Simulation results show that the method of White & Royston (2009) may produce very biased estimates while the CART approach underestimates the imputation uncertainty resulting in low coverage rates. The method of Bartlett et al. (2015) had the best performance overall, with small finite sample bias and coverage rates close to nominal values. We apply the imputation approaches to a Chagas disease dataset.
References
Bartlett, Jonathan W, Seaman, Shaun R, White, Ian R, Carpenter, James R, & Initiative*, Alzheimer’s Disease Neuroimaging. 2015. Multiple imputation of covariates by fully conditional specification: accommodating the substantive model. Statistical methods in medical research, 24(4), 462–487.
Breiman, Leo, Friedman, Jerome H, Olshen, Richard A, & Stone, Charles J. 2017. Classification and regression trees. Routledge.
Carpenter, James, & Kenward, Michael. 2012. Multiple imputation and its application. John Wiley & Sons.
Carpenter, James R., Kenward, Michael G., & Vansteelandt, Stijn. 2006. A comparison of multiple imputation and doubly robust estimation for analyses with missing data. Journal of the Royal Statistical Society: Series A (Statistics in Society), 169(3), 571–584.
Chen, Ming-Hui, Ibrahim, Joseph G, & Shao, Qi-Man. 2009. Maximum likelihood inference for the Cox regression model with applications to missing covariates. Journal of multivariate analysis, 100(9), 2018–2030.
Collins, Linda M, Schafer, Joseph L, & Kam, Chi-Ming. 2001. A comparison of inclusive and restrictive strategies in modern missing data procedures. Psychological methods, 6(4), 330.
Cox, David R. 1972. Regression models and life-tables. Journal of the Royal Statistical Society: Series B (Methodological), 34(2), 187–202.
Cox, David R. 1975. Partial likelihood. Biometrika, 62(2), 269–276.
Hsu, Chiu-Hsieh, & Yu, Mandi. 2019. Cox regression analysis with missing covariates via nonparametric multiple imputation. Statistical methods in medical research, 28(6), 1676–1688.
Kalbfleisch, John D, & Prentice, Ross L. 2011. The statistical analysis of failure time data. Vol. 360. John Wiley & Sons.
Little, Roderick JA, & Rubin, Donald B. 2019. Statistical analysis with missing data. Vol. 793. John Wiley & Sons.
Nunes, Maria Carmo Pereira, Beaton, Andrea, Acquatella, Harry, Bern, Caryn, Bolger, Ann F, Echeverria, Luis E, Dutra, Walderez O, Gascon, Joaquim,
Morillo, Carlos A, Oliveira-Filho, Jamary, et al. 2018. Chagas cardiomyopathy: an update of current clinical knowledge and management: a scientific statement from the American Heart Association. Circulation, 138(12), e169–e209.
Nunes, Maria do Carmo Pereira, Rocha, Manoel Ot´avio C, Ribeiro, Antˆonio Luiz P, Colosimo, Enrico A, Rezende, Renato A, Carmo, Guilherme Augusto A, & Barbosa, Marcia M. 2008. Right ventricular dysfunction is an independent predictor of survival in patients with dilated chronic Chagas’ cardiomyopathy. International journal of cardiology, 127(3), 372–379.
Paik, Myunghee Cho, & Tsai, Wei-Yann. 1997. On using the Cox proportional hazards model with missing covariates. Biometrika, 84(3), 579–593.
Qi, Lihong, Wang, Ying-Fang, & He, Yulei. 2010. A comparison of multiple imputation and fully augmented weighted estimators for Cox regression with missing covariates. Statistics in medicine, 29(25), 2592–2604.
Rathouz, Paul J. 2007. Identifiability assumptions for missing covariate data in failure time regression models. Biostatistics, 8(2), 345–356.
Robins, James M, Rotnitzky, Andrea, & Zhao, Lue Ping. 1994. Estimation of regression coefficients when some regressors are not always observed. Journal of the American statistical Association, 89(427), 846–866.
Rubin, Donald B. 1987. Multiple imputation for survey nonresponse.
Rubin, Donald B. 1996. Multiple imputation after 18+ years. Journal of the American statistical Association, 91(434), 473–489.
Seaman, Shaun R, & White, Ian R. 2013. Review of inverse probability weighting for dealing with missing data. Statistical methods in medical research, 22(3), 278–295.
Seaman, Shaun R, White, Ian R, Copas, Andrew J, & Li, Leah. 2012. Combining multiple imputation and inverse-probability weighting. Biometrics, 68(1), 129–137.
Steyerberg, Ewout W, et al. 2019. Clinical prediction models. Springer.
Tsiatis, Anastasios A. 1981. A large sample study of Cox’s regression model. The Annals of Statistics, 9(1), 93–108.
Van Buuren, Stef. 2018. Flexible imputation of missing data. CRC press.
White, Ian R., & Royston, Patrick. 2009. Imputing missing covariate values for the Cox model. Statistics in medicine, 28(15), 1982–1998.
White, Ian R, Royston, Patrick, & Wood, Angela M. 2011. Multiple imputation using chained equations: issues and guidance for practice. Statistics in medicine, 30(4), 377–399.
Yi, Yanyao, Ye, Ting, Yu, Menggang, & Shao, Jun. 2020. Cox regression with survival-time-dependent missing covariate values. Biometrics, 76(2), 460–471.
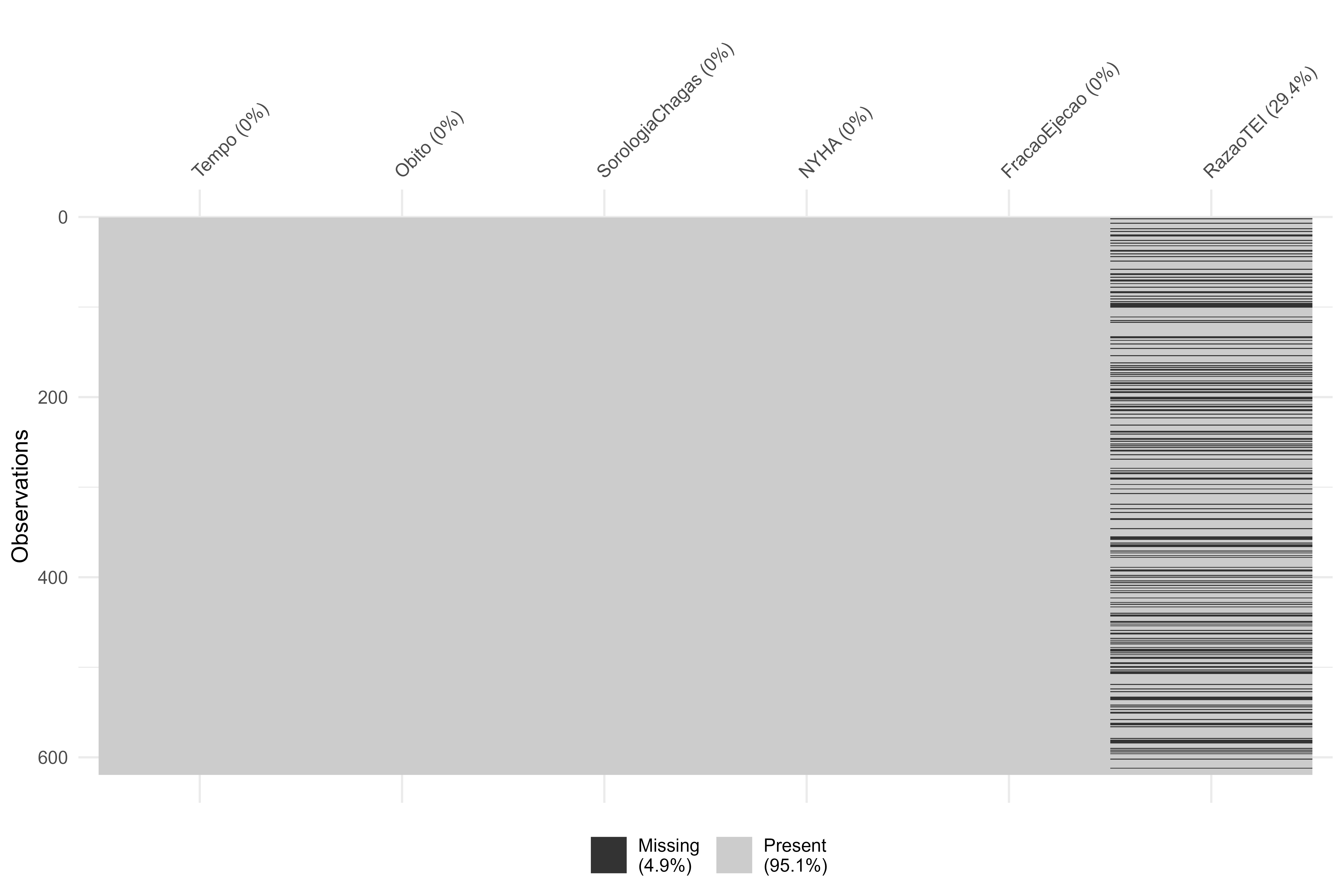
Downloads
Published
How to Cite
Issue
Section
License
Proposta de Política para Periódicos de Acesso Livre
Autores que publicam nesta revista concordam com os seguintes termos:
- Autores mantém os direitos autorais e concedem à revista o direito de primeira publicação, com o trabalho simultaneamente licenciado sob a Licença Creative Commons Attribution que permite o compartilhamento do trabalho com reconhecimento da autoria e publicação inicial nesta revista.
- Autores têm autorização para assumir contratos adicionais separadamente, para distribuição não-exclusiva da versão do trabalho publicada nesta revista (ex.: publicar em repositório institucional ou como capítulo de livro), com reconhecimento de autoria e publicação inicial nesta revista.
- Autores têm permissão e são estimulados a publicar e distribuir seu trabalho online (ex.: em repositórios institucionais ou na sua página pessoal) a qualquer ponto antes ou durante o processo editorial, já que isso pode gerar alterações produtivas, bem como aumentar o impacto e a citação do trabalho publicado (Veja O Efeito do Acesso Livre).