Florianópolis (SC) temperature analysis using a GAMLSS approach
Keywords:
Climate, Meteorology, Distributional regression, VariabilityAbstract
Understanding the variability of climate elements in the temperature is important for economic activities and people's daily lives. With this in mind, the main aim of this paper is to analyse the average temperature of Florianópolis, SC over a one-year period (1 July 2021 to 30 June 2022). The following explanatory variables were considered for this task: date (time), dew point temperature, total precipitation, atmospheric pressure,
humidity, and wind speed. The generalised additive models for location, scale and shape (GAMLSS) were used due to their flexibility to explain the behaviour of the response variable. The Box-Cox power exponential (BCPE) distribution was chosen to explain the response since it can deal with positive variables with varying degrees of kurtosis. A stepwise-based method was performed to select covariates in each of the distribution's parameters. The residuals obtained from the final model were found to be adequate for
explaining the data set.
References
AKAIKE, H. A new look at the statistical model identification. IEEE Transactions on Automatic Control, v. 19, p. 716-723, 1974.
BARLOW, J. W.; CHRISTY, B. P.; O'LEARY, G. J.; RIFFKIN, P. A.; NUTTALL, J. G. Simulating the impact of extreme heat and frost events on wheat crop production: a review. Field Crops Research, v. 171, p. 109--119, 2015.
CEPAGRI/UNICAMP. Escala psicrométrica Unicamp para indicação de níveis de umidade relativa do ar prejudiciais à saúde humana. 2008. Disponível em: urlhttp://www.cpa.unicamp.br/artigos-especiais/umidade-do-arsaude-no-inverno.html
COSTA, A. C. L.; OLIVEIRA, A. D. M.; CARACIOLO, J. P. S.; LUCENA, L. R. R.; LEITE, M. L. M. V. A GAMLSS approach to predicting growth of Nopalea cochenillifera Giant Sweet clone submitted to water and saline stress. Acta Scientiarum. Agronomy, v. 44, p. e54939, 2022.
DUNN, P. K.; SMYTH, G. K. Randomized quantile residuals. Journal of Computational and Graphical Statistics, v. 5, p. 236-244, 1996.
EILERS, P. H. C.; MARX, B. D. Flexible smoothing with B-splines and penalties. Statistical Science, v. 11, p. 89--121, 1996.
GUIMARÃES, P. R. B.; BERGER, R.; PEREZ, F. L.; PIRES, P. T. L. Relações entre as doenças respiratórias e a poluição atmosférica e variáveis climáticas na cidade de Curitiba, Paraná, Brasil. Floresta, v. 42, p. 817--828, 2012.
HERRMANN, M. L. P.; CARDOZO, F. S.; BAUZYS, F.; PEREIRA, G. Freqüência dos desastres naturais no estado de santa catarina no período de 1980 a 2007. In: Encuentro de geógrafos de América Latina, 12. Montevideo, Uruguay. Anais..., 2009. p. 1-12. DVD. Disponível em: urlhttp://urlib.net/ibi/J8LNKAN8RW/36KNHCP.
JARDIM, C. H. Relações entre temperatura, umidade relativa do ar e pressão atmosférica em área urbana: comparação horária entre dois bairros no município de São Paulo-SP. Revista Geografias, v. 7, p. 128--142, 2011.
LEE, J. D.; SUN, D. L.; SUN, Y; TAYLOR, J. E. Exact post-selection inference, with application to the lasso. The Annals of Statistics, v. 44, p. 907--927, 2016.
MURARA, P. G.; MENDONÇA, M.; BONETTI, C. O clima e as doenças circulatórias e respiratórias em Florianópolis/SC. Hygeia, v. 9, 2013.
MENDONÇA, M. A dinâmica têmporo-espacial do clima subtropical na região conurbada de Florianópolis/SC. Tese (Doutorado em Geografia Física). Departamento de Geografia da F.F.L.C.H./USP. São Paulo, 2002.
NAKAMURA, L. R.; CERQUEIRA, P. H. R.; RAMIRES, T. G.; PESCIM, R. R.; RIGBY, R. A.; STASINOPOULOS, D. M. A new continuous distribution on the unit interval applied to modelling the points ratio of football teams. Journal of Applied Statistics, v. 46, p. 416--431, 2019.
NAKAMURA, L. R.; RAMIRES, T. G.; RIGHETTO, A. J.; PESCIM, R. R.; ROQUIM, F. V.; SAVIAN, T. V.; STASINOPOULOS, D. M. Cattle reference growth curves based on centile estimation: A GAMLSS approach. Computers and Electronics in Agriculture, v. 192, p. 106572, 2022a.
NAKAMURA, L. R.; RAMIRES, T. G.; RIGHETTO, A. J.; SILVA, V.; KONRATH, A. C. Using the Box-Cox family of distributions to model censored data: a distributional regression approach. Brazilian Journal of Biometrics, v. 40, p. 407-414, 2022b.
NAKAMURA, L. R.; RIGBY, R. A.; STASINOPOULOS, D. M.; LEANDRO, R. A.; VILLEGAS, C.; PESCIM, R. R. Modelling location, scale and shape
parameters of the Birnbaum-Saunders generalized t distribution. Journal of Data Science, v. 15, p. 221--237, 2017.
RAMIRES, T. G.; NAKAMURA, L. R.; RIGHETTO, A. J.; CARVALHO, R. J.; VIEIRA, L. A.; PEREIRA, C. A. B. Comparison between highly complex location models and GAMLSS. Entropy, v. 23, p. 469, 2021a.
RAMIRES, T. G.; NAKAMURA, L. R.; RIGHETTO, A. J.; KONRATH, A. C.; PEREIRA, C. A. B. Incorporating clustering techniques into GAMLSS. Stats, v. 4, p. 916--930, 2021b.
RAMIRES, T. G.; NAKAMURA, L. R.; RIGHETTO, A. J.; ORTEGA, E. M. M.; CORDEIRO, G. M. Predicting survival function and identifying associated factors in patients with renal insufficiency in the metropolitan area of Maringá, Paraná State, Brazil. Cadernos de Saúde Pública, v. 34, p. e00075517, 2018.
RAMIRES, T. G.; NAKAMURA, L. R.; RIGHETTO, A. J.; PESCIM, R. R.; MAZUCHELI, J.; CORDEIRO, G. M. A new semiparametric Weibull cure rate model: fitting different behaviors within GAMLSS. Journal of Applied Statistics, v. 46, p. 2744--2760, 2019.
RAMIRES, T. G.; NAKAMURA, L. R.; RIGHETTO, A. J.; PESCIM, R. R.; MAZUCHELI, J.; RIGBY, R. A.; STASINOPOULOS, D. M. Validation of stepwise-based procedure in GAMLSS. Journal of Data Science, v. 19, p. 96--110, 2021c.
RIGBY, R. A.; STASINOPOULOS, D. M. Smooth centile curves for skew and kurtotic data modelled using the Box-Cox power exponential distribution. Statistics in Medicine, v. 23, p. 3053-3076, 2004.
RIGBY, R. A.; STASINOPOULOS, M. D.; HELLER, G. Z.; DE BASTIANI, F. Distributions for Modeling Location, Scale, and Shape: Using GAMLSS in R. Boca Raton: CRC Press. 2019. 560 p.
RIGHETTO, A. J.; RAMIRES, T. G.; NAKAMURA, L. R.; CASTANHO, P. L. D. B.; FAES, C.; SAVIAN, T. V. Predicting weed invasion in a sugarcane cultivar using multispectral image. Journal of Applied Statistics, v. 46, p. 1--12, 2019.
SILVA, E. M.; ASSUNÇÃO, W. L. O clima da cidade de Uberlândia-MG. Sociedade & Natureza, v. 16, p. 91--107, 2004.
STASINOPOULOS, M. D.; RIGBY, R. A.; HELLER, G. Z.; VOUDOURIS, V.; DE BASTIANI, F. Flexible Regression and Smoothing: Using GAMLSS in R. Boca Raton: CRC Press. 2017. 572 p.
TALAIA, M.; VIGÁRIO, C. Temperatura de ponto de orvalho: um risco ou uma necessidade, 2016. Disponível em: urlhttp://hdl.handle.net/10316.2/39909.
VAN BUUREN, S.; FREDRIKS, M. Worm plot: a simple diagnostic device for modelling growth reference curves. Statistics in Medicine, v. 20, p. 1259-1277, 2001.
WMO (World Meteorological Organization). Guide to meteorological instruments and methods of observation, 2008.
YAMADA, G.; JONES-SMITH, J. C.; CASTILLO-SALGADO, C.; MOULTON, L. H. Differences in magnitude and rates of change in BMI distributions by socioeconomic and geographic factors in Mexico, Colombia, and Peru, 2005--2010. European Journal of Clinical Nutrition, v. 74, p. 472--480, 2020.
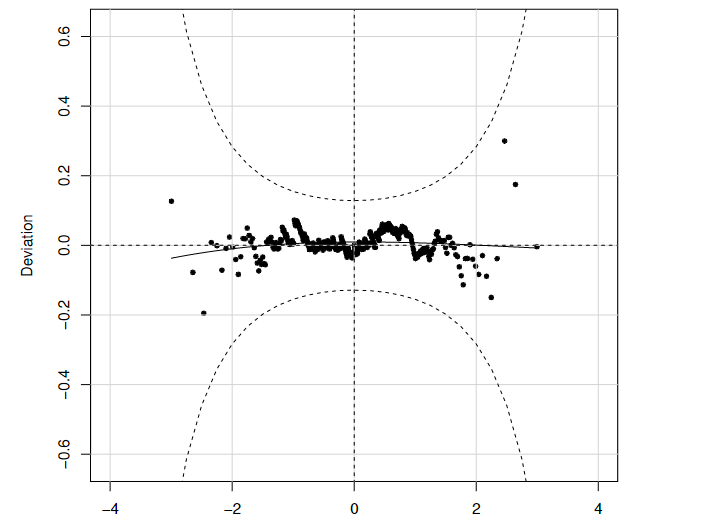
Downloads
Published
How to Cite
Issue
Section
License
Proposta de Política para Periódicos de Acesso Livre
Autores que publicam nesta revista concordam com os seguintes termos:
- Autores mantém os direitos autorais e concedem à revista o direito de primeira publicação, com o trabalho simultaneamente licenciado sob a Licença Creative Commons Attribution que permite o compartilhamento do trabalho com reconhecimento da autoria e publicação inicial nesta revista.
- Autores têm autorização para assumir contratos adicionais separadamente, para distribuição não-exclusiva da versão do trabalho publicada nesta revista (ex.: publicar em repositório institucional ou como capítulo de livro), com reconhecimento de autoria e publicação inicial nesta revista.
- Autores têm permissão e são estimulados a publicar e distribuir seu trabalho online (ex.: em repositórios institucionais ou na sua página pessoal) a qualquer ponto antes ou durante o processo editorial, já que isso pode gerar alterações produtivas, bem como aumentar o impacto e a citação do trabalho publicado (Veja O Efeito do Acesso Livre).